Jun 01, 2021
LWM successfully participates in the PHME21 Data Challenge
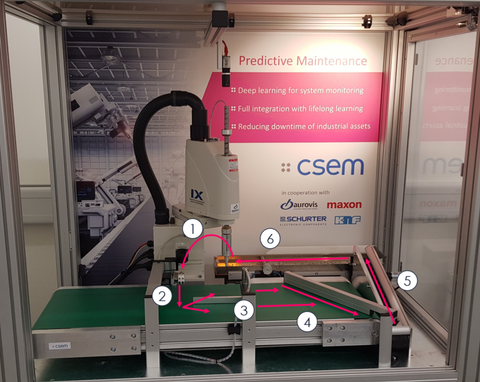
Fig. 1: Test stand with a robotic system at the PHME21 Data Challenge
"Are the models correct? Are the error classes predicted correctly? Are the predictions fast enough? How do we do against the other teams?" These were the thoughts running through the minds of the LWM staff late at night – just before submitting their entry to this year's PHME21 (Prognostics and Health Management Societey Europe) Data Challenge. The questions have now been officially answered by PHME. As Team PrIMA (derived from the research department ProcessInformatics and MachinedataAnalysis), our employees Felix Conrad, Kim Feldhoff, Mauritz Mälzer and Dr. Michael Schwarzenberger took part in a Data Challenge for the first time and were immediately successful with 6th place out of a total of 21 participating teams. International teams from industry and research were at the start.
For more than 10 years, a Data Challenge has been held in the run-up to the PHME conference to develop systems for detecting and predicting faults and damage. In this worldwide competition to find the best solution, each time there is a new problem with special challenges.
This year, the task was related to a test stand for the automatic testing of electrical fuses. A condition monitoring system was to be developed for this purpose. The test stand included a robot arm, two camera systems and several conveyor belts. In this system, eight unknown fault classes and normal operation were to be detected. The faults were systematically installed by the PHME, e.g. the exposure of one camera was manipulated. To make it even more difficult, normal operation was divided into two unknown operation modes. The data set provided contained over 50 signals, including machine behavior (e.g., pressure, vacuum, speed), environment (e.g., temperature and humidity), and other aspects (e.g., CPU temperature, memory utilization). Based on the data set, the failure classes were to be predicted as quickly as possible. In addition, a justification for the respective error classification was required. Thus, it was necessary to find out which signals were responsible for the predicted error.
The Data Challenge required a solution that went far beyond the blind application of AI procedures. Among other things, in-depth process knowledge played an important role in correctly identifying errors and distinguishing important from unimportant signals. Some faults, such as a leaking vacuum gripper valve, were easier to identify. Other errors, such as incorrect adjustment of the robot movements, were more difficult to identify. Here, methods of ExplainableML, i.e. explainable machine learning, helped us. In the end, the LWM team was able to use detailed exploratory data analysis and tree-based ML methods to reliably predict both of the unknown modes of normal operation as well as seven of the eight fault classes.
Participation in the Data Challenge led to intense collaboration within the team. In the process, the Data Challenge quickly developed into an ambitious recreational project. Everyone continuously contributed new ideas and methods to make the predictions even faster and more stable. This effort paid off – on the one hand because of the good ranking, on the other hand because of the great increase in knowledge. The newly applied software tools and prediction methods and the knowledge gained are now benefiting the research projects at LWM, among others.
More information about the Data Challenge can be found at https://phm-europe.org/data-challenge. The full final result (team name "PriMA") can be viewed on the PHME website: https://phm-europe.org/data-challenge-results
LWM / Process Informatics and Machine Data Analysis / Dr. Michael Schwarzenberger