Research topics
Research topics
The research of the Envrionmental Remote Sensing Group focuses on the application and development of satellite data sets and derived products to observe, analyse, model and predict changes in vegetation, fires and the related impacts on the carbon and water cycle in ecosystems. We use and develop data-driven methods such as time series analysis and machine learning as well as process-based environmental models and model-data integration techniques.
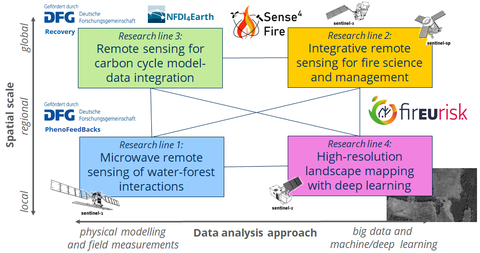
Overview of the four main research lines with associated third-party funded projects and the satellites used from the European Copernicus programme.
The research in the EnvRS group is clustered in four inter-linked research lines. The four research lines cover different scales (local to global) and make use of different approaches of data analysis in remote sensing (e.g. field measurements, physical modelling, radiative transfer modelling, model inversion, time series analysis, machine learning, deep learning). Each research line addresses specific objectives that link technical remote sensing-related aspects with environmental sciences:
- Microwave remote sensing of water-forest interactions: The objective is to develop an understanding of the sensitivity and physical relations of active and passive microwave observations to leaf moisture content, vegetation water content, canopy interception and vegetation phenology. This research enables to quantify the amount of water in terrestrial vegetation from microwave satellite observations and to assess the impact of such estimates on various ecosystem processes such as interception, plant productivity and fire danger.
- Integrative remote sensing for fire science and management: The objective is to combine several observations from optical and microwave remote sensing using various satellite sensors and products and to develop integrative methods in order to quantify and monitor various aspects of vegetation fires such as fuel loads, fire danger, the development of fires and emissions of smoke and greenhouse gases. We develop and apply machine learning methods to predict fire ignitions and fire danger. Within this research line, we also closely cooperate with practitioners from fire brigades, forestry and public administrations in order to reduce current and to prepare for future wildfire risks.
- Remote sensing for carbon cycle model-data integration: The objective is to exploit the wealth of satellite observations and to develop related methods to calibrate, develop and evaluate global ecosystem carbon cycle and Earth system models. Remote sensing-based carbon cycle model-data integration advances the understanding and quantification of changes in climate and extreme events such as drought effects on ecosystem carbon stocks and fluxes, the global carbon cycle and on potential tipping elements in the climate system.
- High-resolution landscape mapping with deep learning: The objective is to adapt, develop, and apply methods from AI/deep learning to map land cover, land use or structural elements in landscapes with high resolution aerial and satellite imagery. This information is used to assess biodiversity and ecosystem services. A unique focus of the research is the use of historical high-resolution Earth observation imagery such as from the “Corona” spy programme of the CIA from the 1960s and 1970s.