Jul 24, 2024
Deep learning application reveals new insights into the dynamics of Greenland outlet glaciers
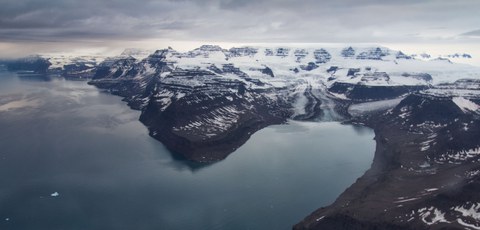
Marine-terminating outlet glaciers in Greenland (Scoresby Sund)
From 1992 to 2020 the Greenland Ice Sheet has lost 4892±457 Gt of ice, raising the global sea level by 21±2 mm. Models suggest that this imbalance will continue with a warming climate. The mass balance is strongly influenced by the outlet glaciers through which ice masses are discharged into the ocean. A key parameter for investigating the mechanisms of these glaciers is the variation of their calving front.
Comprehensive datasets of calving front changes are therefore essential not only for studying outlet glaciers, but also for constraining ice sheet models and improving simulations of future mass loss and sea level contribution. However, current records are limited in temporal resolution due to manual delineation which is a very laborious and time-consuming process.
AI for automated calving front delineation
We apply artificial intelligence methods, specifically deep learning, to automatically delineate glacier calving fronts from optical satellite imagery. The core of our workflow builds on recent advances in the field of deep learning while taking full advantage of multispectral input information. Automatically extracted calving fronts are of comparable quality to manually delineated fronts. The fully automated working method enables us to provide comprehensive data sets for all important outlet glaciers in Greenland.
Long-term, seasonal, and subseasonal calving front changes
The resulting data product includes 9242 calving fronts over 23 Greenland glaciers.
While long-term changes are easy to resolve using already available data products, our time series offers unique opportunities to analyze seasonal and subseasonal terminus changes. Depending on the weather and season, glaciers are observed up to six times per week. As an example, Figure 2 shows such a time series for Jakobshavn Isbræ (western Greenland), which is divided into a northern and a southern branch. The inferred calving front variation shows a pronounced annual pattern combined with smaller subseasonal variations.
The capability of automatically resolving subseasonal calving front variations is an important step towards having a spatially comprehensive Greenland-wide monitoring system. In conjunction with other components concerning ice flow, elevation change, solid-Earth response, and hydrological processes, this will open up new opportunities to holistically assess, model, and simulate dynamic ice sheet changes.
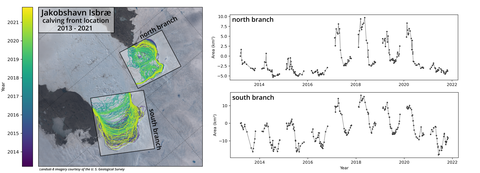
AI-generated calving front time series for Jakobshavn Isbræ (western Greenland). The glacier, which is separated into a northern branch and a southern branch, and the calving fronts are shown on the left. The corresponding time series are depicted on the right. Here, calving front positions, expressed as surface areas, are marked by connected dots.
Interested in details?
For more information, have a look at the full paper "Calving front monitoring at a subseasonal resolution: a deep learning application for Greenland glaciers".
The study, led by Erik Loebel, was recently published in the journal "The Cryosphere".
Loebel, E., Scheinert, M., Horwath, M., Humbert, A., Sohn, J., Heidler, K., Liebezeit, C., and Zhu, X. X.: Calving front monitoring at a subseasonal resolution: a deep learning application for Greenland glaciers, The Cryosphere, 18, 3315–3332, https://doi.org/10.5194/tc-18-3315-2024, 2024.
This research is a collaboration with partners from the Alfred Wegener Institute, Helmholtz Centre for Polar and Marine Research and the Technische Universität München, Chair of Data Science in Earth Observation. This work was supported by the Helmholtz Association of German Research Centers as part of the Helmholtz Information & Data Science pilot project “Artificial Intelligence for Cold Regions” (AI-CORE) and by the German Federal Ministry of Education and Research (BMBF) project “Greenland Ice Sheet/Ocean Interaction” (GROCE2).
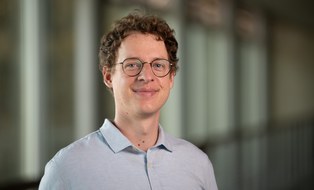
Research Associate
NameErik Loebel M.Sc.
Send encrypted email via the SecureMail portal (for TUD external users only).