May 09, 2023
Understanding Complex Systems Like the Brain by Analysing the Hidden Geometry of Their Networks
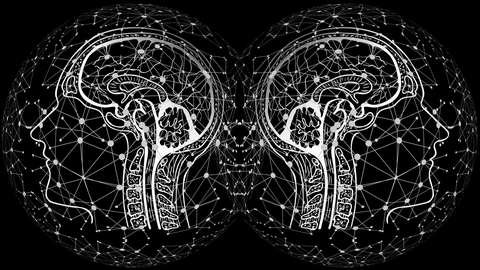
An artistic representation of brain networks.
A study published in the journal Nature Communications and led by Prof. Carlo Vittorio Cannistraci, Director of the Center for Complex Network Intelligence (CCNI) at the Tsinghua Laboratory of Brain and Intelligence and Biotechnology Center (BIOTEC) of TU Dresden alumnus, proposes a fast algorithm to measure the relationship between the spatial variables of an interconnected complex system, its geometry and its navigability. The new algorithm can help to enhance our understanding of the brain differences across age and sex.
Many variables shape the connectivity of networks associated with complex systems such as the human society. Some of these variables are known such as the higher the popularity of a person, the greater the number of connections that person can have in social media networks. Another example are variables such as semantic or spatial proximity. The more people share common interests or live in geographic proximity, the more likely they are to connect and form small and medium-sized communities in a network. If variables such as popularity and proximity are known, many other variables that shape the connectivity of the network in a complex system are unknown. However, the geometric relationship of these variables in a multidimensional space, which scientists call manifold, remains imprinted as a “trace” in the network structure.
In their recent publication in Nature Communications Prof. Cannistraci and Dr. Muscoloni developed and implemented an optimized algorithm that reduces the worst-case scenario computation time from 26 years to just one week. The algorithm is able to measure the extent to which a network topology matches with an associated manifold geometry. The manifold represents the rule of geometric associations between all the variables that contribute to forming the shape of the network in a complex system, and the network is a kind of discretization of this manifold. The hidden geometry of the manifold behind the structure of brain networks is unknown.
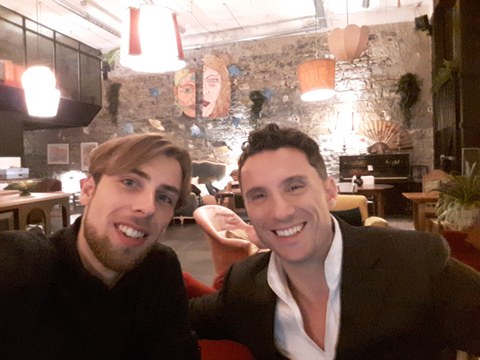
Portrait: Dr. Alessandro Muscoloni (on the left) and Prof. Carlo Vittorio Cannistraci (on the right).
“Although we visualize brain networks in a three-dimensional space, the number of variables that shape their architecture is higher. Some of these variables such as age and sex are known, but many others are unknown, nevertheless, we can still find their trace in the shape of the brain network, therefore we can try to measure how congruent is the shape of a network with its hidden geometry. This measure can be used as a marker that can differentiate different states or conditions of brain networks, and therefore can help to envision new theories and measures to design markers for brain diseases,” says Prof. Cannistraci together with his colleague Dr. Alessandro Muscoloni.
Carlo Vittorio Cannistraci led the Biomedical Cybernetics group at the Biotechnology Center (BIOTEC) of TU Dresden, where he started this study together with Dr. Alessandro Muscoloni, who was a post-doc in Cannistraci’s team at the time. Two years ago, Carlo Vittorio Cannistraci moved to the Tsinghua University in China, where he is Chair Professor and Director of the Centre for Complex Network Intelligence.
“If physics studies the principles and mechanisms of the outside universe, brain science studies the principles and mechanisms of the inside universe. My research is at the interface between these two disciplines. I deal with physics and engineering of complexity and intelligence: studying principles of natural and artificial intelligence,” explains Prof. Cannistraci.
Publication:
Carlo Vittorio Cannistraci and Alessandro Muscoloni: Geometrical congruence, greedy navigability and myopic transfer in complex networks and brain connectomes. Nature Communications (November 2022).
Link: https://doi.org/10.1038/s41467-022-34634-6
Scientific contact:
Prof. Carlo Vittorio Cannistraci
Email:
Tel: +8613522941320, +39 3475954094