Research Data Management (RDM) - A Practical Solution for the Acquisition, Integration and Analysis of Research Data in Collaborative Engineering Projects
Motivation
Nowadays, data-driven methods are increasingly used in collaborative engineering projects to build up knowledge about interactions between material properties, process influences, structural parameters and product properties. The required data sets usually originate from different data sources (processes, systems, devices, software, etc.) and have to be made accessible by means of digitization. Due to the manifold influences, e.g. on the material behavior or due to the acting interactions in the manufacturing processes, cooperations between the relevant engineering disciplines are usually required. This typically results in several partners being involved in the data acquisition in the joint project. Different partners have different working cultures, different technical languages, different technical infrastructures.
Research data management (RDM) is a mandatory prerequisite for the actual task of generating and modeling knowledge by means of data analysis. The working group "Machine Data Exploitation" has expertise in data analytics and is therefore often involved in collaborative projects. There, however, analyzable data sets must usually first be created from the partners' individual data, which requires a well-founded concept for RDM, including implementation. Figure 1 summarizes the requirements that we consider important for a practicable RDM.
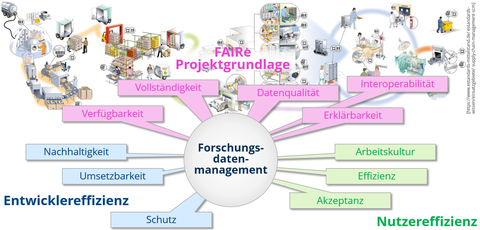
Challenges for RDM
Figure 1: The view of the Research Group „Machine Data Processing“ of the Challenges for a practicable RDM [1]
Goal of research and development
The goal of our work is to develop a suitable concept for RDM for collaborative projects, especially in the engineering sciences. The focus is on the sustainable documentation of research data in compliance with the FAIR principles as well as the linking of data along the process chain and finally the establishment of the analyzability of data. Since a successful RDM lives on the acceptance of the RDM system by the users, all supporting functions that improve the users' work with RDM and beyond are equally important to us.
Challenges for a practicable FDM [2]
required data flows and to enable consortia to collaborate. Both classic requirements such as the FAIR principles (F: Findable: A: Accessible, I: Interoperable: R: Reusable) with regard to research data and project-specific requirements must be taken into account. In sum, the requirements for the RDM and the research data infrastructure (RDI) are usually as follows (Figure 1):
FAIR principles
- Good accessibility of data (internal and external accessibility of data) is needed so that work can be done from different locations.
- High data protection is needed to protect research data from unauthorized access. In particular, this requires fine-grained role rights management and encrypted connections in the RDI.
- Easy discoverability or good searchability of data is needed for efficient work in the collaborative project.
- Good interoperability of data is needed for linking datasets from different processes (involvement of different labs and different project partners).
- Sustainability of the data is needed for the use of the data during and after the end of the project by archiving the data.
- Citeability of the data is needed for referencing published research data, e.g. via persistent identifiers such as Digital Object Identifier (DOI).
Documentation of the data
- Comprehensible documentation and good explicability of the data is needed so that subject matter experts involved in the project and third parties understand what the data mean and under what objectives and conditions they were generated. For a comprehensible documentation of experiments it is necessary that used procedures, machines, tools and materials are described in detail to be able to establish correlations. The descriptions should be based on a common technical language so that all researchers in the joint project, who often come from different disciplines, understand the same terms.
- Unambiguous designation of samples is necessary for merging data from different processes and for tracing samples along process chains.
- Linking of data along the process chain is needed for exploring cross-process interactions
Practicability
- High sustainability and flexibility of the RDM concept are essential for the transfer and further development of the RDM concept to other collaborative projects. In particular, it should be possible to incorporate current developments from the NFDI research data initiative.
- Low effort for setup and maintenance of the RDI are crucial for a fast implementation of the FDM concept and the workability of the consortium.
- High usability of the RDI is necessary for high adoption of the system to get researchers motivated to use the RDM.
- Retention or integration of working cultures of the participating laboratories is necessary to ensure acceptance of the RDM concept and to avoid parallel worlds or laboratory-owned solutions.
Sustainable use
- Implementing the FAIR principles in a viable RDM usually has to be done with low capacity. Therefore, the RDM solution should be sustainable (reusable and adaptable) for subsequent projects.
Our solution approach, our architecture of the FDM [2]
RDM concept and basic functionality
- Separation of data storage (via file system) and data management (via web frontend) for independent access
- Secure data exchange through encrypted connections (SSH, HTTPS)
- Fine-grained rights management in the web frontend and in data storage through ID management
- Access to research data for external project partners (institutes, companies) via VPN
- Documentation of research data via metadata schemas
- Subject-specific taxonomy for keywording and for use in metadata schemas
Functions for practicability
- Data management via familiar and familiar web interface (MS SharePoint site)
- Agile sample ID management
- Mapping of sample flow / traceability of samples via linking of sample IDs
- Adaptation of keywording to common technical language by linking to ontology for applied sciences (here: EMMO: European Materials Modeling Ontology)
- Data and computationally intensive analyses & simulations by using HPC resources
- Continuous workflow from data acquisition to data publication (via DOI)
Efficient and sustainable RDM solution
- Use of IT infrastructure and service offerings of the data center (here: ZIH of TU Dresden)
- Use of basic functions in MS SharePoint
- Easy transferability to new engineering projects
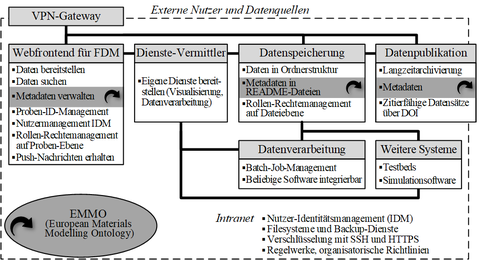
Architecture for the practicable RDM system of the Work group "Machin data processing"
Figure 2. Architecture for the practicable RDM system [2]
Current research
- Generalization and review of prototypical functions for easy deployment to other projects and to other partners
- Automated tagging
- Navigation in the data via graphical process views that correspond to the workflows in the project.
Call to Action
We are interested in exciting research questions that we can answer in a data-driven way. We bring the expertise to a viable RDM and serve as an interface to IT departments.
We are interested in the after-use of our RDM solution and in being able to further develop it according to their requirements.
We are happy to conduct workshops on FDM, especially for engineering projects.
References
5 |
Zinner, M.; Conrad, F.; Feldhoff, K.; Wiemer, H.; Weller, J.; Ihlenfeldt, S.: A Metadata Model for Harmonising Engineering Research Data Across Process and Laboratory Boundaries. in COGNTIVE 2024: The Sixteenth International Conference on Advanced Cognitive Technologies and Applications, 2024, pp. 30-39. [Online]. Available: https://www.thinkmind.org/articles/cognitive_2024_1_50_40019.pdf |
4 | Raßloff, Alexander; Feldhoff, Kim; Wiemer, Hajo; Zimmermann, Martina; Kästner, Markus: AMTwin - Datengetriebene Prozess-, Werkstoff- und Strukturanalyse für die additive Fertigung. Konferenzbeitrag „Mobilität der Zukunft – Bauteilzuverlässigkeit im digitalen Zeitalter - DVM-Tag 2023“, Berlin, 2023, http://doi.org/10.48447/DVM-TAG-2023-150 |
3 | Wiemer, Hajo; Feldhoff, Kim; Ihlenfeldt, Steffen: Praktikable IT-Infrastruktur zur Erfassung, Integration und Analyse von Forschungsdaten in Ingenieursverbundprojekten. Poster „SaxFDM-Tagung“, 2022, Zenodo. http://doi.org/10.5281/ZENODO.7155861 |
2 | Feldhoff, K. ; Wiemer, H. : Praktikables, Ontologie-basiertes Forschungsdatenmanagement in der Additiven Fertigung. In: Brockmann, S. ; Krupp, U. (Hrsg.): 39. Vortrags- und Diskussionstagung Werkstoffprüfung „Werkstoffe und Bauteile auf dem Prüfstand: Prüftechnik – Kennwertermittlung – Schadensvermeidung“. Düsseldorf: Stahlinstitut VDEh, 2021, ISBN 978-3-941269-98-9 |
1 | Feldhoff, K. ; Wiemer, H. ; Ihlenfeldt, S. : FDM als Service für ein typisches Verbundprojekt in den Ingenieurwissenschaften auf Basis einer ontologie-basierten Verschlagwortung. In: Digital Kitchen von SaxFDM, online, 18.11.2021 DOI: 10.5281/zenodo.5718660 |