Mini Courses
Building Trust in AI: Alstom’s Journey Towards New, Safe, and Reliable Mobility Solutions
Nenad Mijatovic, Ph.D.,Chief AI & Data Scientist at Alstom
As aspiring professionals in the rail industry, you are entering a field ripe for transformation through technology. While AI applications in mobility have largely focused on non-safety-critical aspects, we stand on the brink of a revolution in safety-critical applications such as autonomous trains and advanced signalling systems. In this session, Nenad will explore how Alstom is leading the charge in building trust in AI. He will discuss the critical importance of integrating AI safely into safety-critical systems and share innovative use cases tailored for the rail sector. Learn about an AI solution that detects failures in radio communication—vital for safe train operations—and discover how smart troubleshooting systems leverage predictive maintenance and human-centric AI technologies, including Large Language Models (LLMs), to empower engineers in swiftly diagnosing and resolving system issues. Join us for an interactive session that not only showcases Alstom's commitment to trustworthy AI solutions but also prepares you for the exciting advancements that lie ahead in the rail industry. Together, we can harness the power of AI to shape a safer and more efficient future for mobility.
CV of Nenad Mijatović:
Nenad has over 20 years of experience in algorithm development, machine learning, and AI, and has been active in the rail industry since 2014, giving him more than a decade of specialized expertise in this field. Throughout his career, he has held various R&D and leadership positions in startups, blue-chip companies, and academia. As the Chief AI & Data Scientist, Nenad leads data science teams in the development of Machine Learning and AI algorithms for various mobility solutions. The team tackles mobility challenges in both urban and rural areas, emphasizing innovation, efficiency, and sustainability to support railway operators in achieving their operational objectives. In his free time, Nenad enjoys reading science fiction, philosophical, and historical books, playing tennis, and appreciating art. He is also passionate about promoting math and computer science skills among girls and young women, inspiring them to pursue technical degrees.
Energy efficient train operation
Pengling Wang, Tongji
This mini-course presents the fundamental theories, state-of-the-art models and algorithms for the energy efficient train operation. Energy and environmental sustainability in transportation have received increasing attention in recent decades. Railway, as one of the most energy-efficient transport, plays an essential role in improving the world’s energy and environmental sustainability. The energy consumption of a railway system is composed of many parts, among which, traction energy consumption accounts for about 80% of the total energy consumption of the railway system, which is the main focus of energy conservation research. In this mini-course, we will focus on the fundamental methods for saving traction energy consumption and improving the usage of regenerative energy. The course discusses timely topics such as energy-efficient train trajectory optimization, energy-efficient timetable optimization, regenerative braking energy utilization improvement, integrated train rescheduling and train control, etc. The course also presents a few research case-studies to demonstrate concepts and showcase potential applications.
CV of Pengling Wang: Wang Pengling, female, born in November 1989, is currently an Associate Professor at the School of Transportation, Tongji University. She obtained her Ph.D. in 2017 from Delft University of Technology and conducted postdoctoral research at ETH Zurich from 2018 to 2021. Her main research areas include train operation optimization and control, intelligent transportation organization and management for urban rail transit. She is currently serving as an associate editor for IET ITS and a young editorial board member for the Journal of Railway Science and Engineering. She has been selected for several prestigious programs, including the "Rubicon Scholars" program of the Netherlands Organization for Scientific Research, the "Shanghai High-Level Talent Introduction" program in China, and the "Alexander von Humboldt Fellow" program in Germany. She has led multiple provincial and national-level research projects, as well as several industry-sponsored projects, and has published over 40 papers, several of which have been published in high-impact journals such as TR-C, TR-B, IEEE ITS etc.
Spatio-temporal networks for mapping railway operations
Deb Panja, Utrecht University
Propagation of delays in railway systems — a complex system — is an emergent phenomenon that vary dramatically over space and time, making it challenging to predict future system trajectories at “macro”, system-level scales. Spatio-temporal variations in delays at the macro-scale stem from spatial and temporal heterogeneities in their generating mechanisms at the ``micro'' scales; i.e., from the heterogeneities in the location, timing, and nature of physical interactions among system actors: scheduled services, rolling stocks and crew in the case of railways. We develop spatio-temporal networks as a details-driven and data-compatible framework for unravelling spatio-temporal variations in emergent dynamics of real-world complex systems, and use railways as an application area to demonstrate the efficacy of the framework: which bottlenecks in a railway system can be anticipated. We will demonstrate how the framework may prove to be significantly advantageous in unravelling spatio-temporal development of delays in railway systems.
CV of Deb Panja: Dr. Panja specialises in developing analytical/computational methods that are rooted in various naturally occurring as well as engineered complex systems, with specific focus on dynamical systems, (multi-scale) agent-based models and networks aspects, and correspondingly, concepts and models to unravel real-world phenomena in a wide variety of disciplines, e.g., on social networks, economic complexity, neuroscience, transport, human behaviour, ecosystems, epidemiology, and materials and fluids. He is the vice-director of the Centre for Complex Systems Studies at Utrecht University.
Challenges and solutions of solving a large-scale networked high-speed railway disruption management problem
by Professor Lingyun Meng & Dr. Zhengwen Liao, Beijing Jiaotong University, China
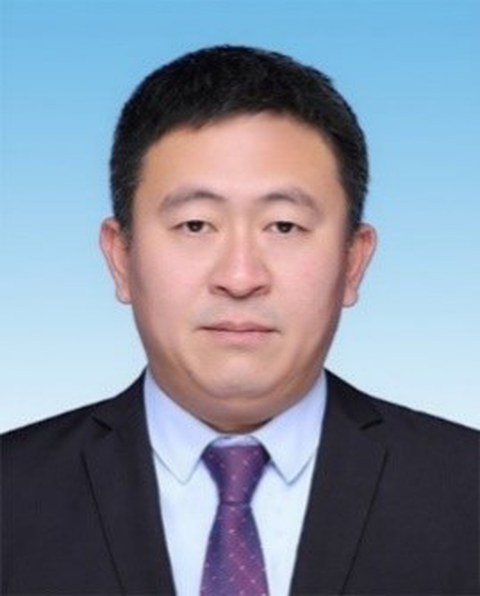
Prof. Dr. Lingyun Meng
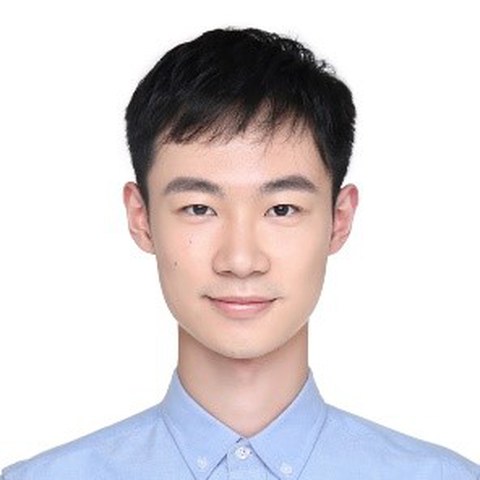
Dr. Zhengwen Liao
In high-speed railway operations, unavoidable perturbations such as infrastructure failures and adverse weather conditions often occur, leading to delays and even service cancellations. Effective railway traffic management is essential for minimizing these impacts and improving service punctuality and reliability. This requires efficiently rescheduling trains while managing various resource constraints such as tracks and rolling stock, ensuring smooth and reliable operations even in the face of unforeseen disruptions. This lecture introduces a multi-solution approach to solve multi-granularity problems in high-speed railway disruption management. We examine tailored solutions to three key challenges: 1) complex railway terminals; 2) dense railway corridors; and 3) large-scale railway networks. By applying advanced optimization models and strategies, the lecture will demonstrate how these solutions can enhance system efficiency during disruptions. The conclusions will highlight the practical relevance and real-world applicability of these solutions in improving railway operations.
CV of Lingyun Meng: Lingyun Meng is a professor, the dean of the School of Traffic and Transportation, at Beijing Jiaotong University. His research interests include railway traffic management, disruption management, rolling stock management, and the integration of advanced technologies such as human-computer interaction in railway operations. He is actively involved in both academic and applied research, frequently presenting his work at international conferences and publishing in top-tier journals. He has developed an intelligent train dispatching decision engine and a train conflict detection and resolution system for hub stations. These systems have been successfully implemented at various railway bureaus, including those in Beijing, Shenyang, Chengdu, and Hohhot.
CV of Zhengwen Liao: Zhengwen Liao is a lecturer at the School of Traffic and Transportation of Beijing Jiaotong University. His research interests include railway capacity estimation, network-wide large-scale train rescheduling models, algorithms, and their application in real-world environments. With tight cooperation with China Railway, the algorithm modules he develops are embedded into the online dispatching system to provide decision support. As the primary investigator, he is in charge of the NSFC project entitled Hierarchical asynchronous collaborative optimization method for train rescheduling on high-speed railway network.