Dipl.-Ing. Lennart Linden
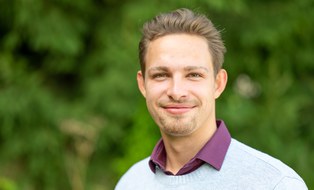
Dipl.-Ing. Lennart Linden
Professur für Numerische und Experimentelle Festkörpermechanik
Professur für Numerische und Experimentelle Festkörpermechanik
Besuchsadresse:
Zeunerbau, Zimmer 350 George-Bähr-Straße 3c
01069 Dresden
Forschung
- Datengetriebene Materialmodellierung und Simulationstechniken
- Anwendung neuronaler Netze in der Festkörpermechanik
- Einbettung physikalischer Grundprinzipien in neuronale Netze
- ResearchGate, GoogleScholar
Lehre
- Übungsbetreuung Kontinuumsmechanik (Hauptstudium)
- Übungsbetreuung Finite-Elemente-Methode (Hauptstudium)
- Übungsbetreuung Statik (Grundstudium)
Publikationen
2024
-
Neural network-based multiscale modeling of finite strain magneto-elasticity with relaxed convexity criteria , März 2024, in: Computer Methods in Applied Mechanics and Engineering. 421, 116739Elektronische (Volltext-)VersionPublikation: Beitrag in Fachzeitschrift > Forschungsartikel
2023
-
Neural networks meet hyperelasticity: A guide to enforcing physics , Okt. 2023, in: Journal of the Mechanics and Physics of Solids. 179, 105363Elektronische (Volltext-)VersionPublikation: Beitrag in Fachzeitschrift > Forschungsartikel
-
FEANN: an efficient data-driven multiscale approach based on physics-constrained neural networks and automated data mining , 8 Feb. 2023, in: Computational Mechanics : solids, fluids, engineered materials, aging infrastructure, molecular dynamics, heat transfer, manufacturing processes, optimization, fracture & integrity. 71, 5, S. 827-851, 25 S.Elektronische (Volltext-)VersionPublikation: Beitrag in Fachzeitschrift > Forschungsartikel
2022
-
FEANN - An efficient data-driven multiscale approach based on physics-constrained neural networks and automated data mining , 3 Juli 2022, 22 S.Elektronische (Volltext-)VersionPublikation: Vorabdruck/Dokumentation/Bericht > Vorabdruck (Preprint)
-
Automated constitutive modeling of isotropic hyperelasticity based on artificial neural networks , Jan. 2022, in: Computational Mechanics : solids, fluids, engineered materials, aging infrastructure, molecular dynamics, heat transfer, manufacturing processes, optimization, fracture & integrity. 69, S. 213-232, 20 S.Elektronische (Volltext-)VersionPublikation: Beitrag in Fachzeitschrift > Forschungsartikel
2021
-
Thermodynamically consistent constitutive modeling of isotropic hyperelasticity based on artificial neural networks , 2021, in: Proceedings in Applied Mathematics and Mechanics: PAMM. 21, 1, S. e202100144, 3 S.Elektronische (Volltext-)VersionPublikation: Beitrag in Fachzeitschrift > Konferenzartikel
Konferenzbeiträge
-
L. Linden, K. A. Kalina, J. Brummund, M. Kästner, Automated constitutive modeling of isotropic hyperelasticity based on artificial neural networks, 91st Annual Meeting of the International Association of Applied Mathematics and Mechanics, Online, 2021
-
L. Linden, K. A. Kalina, J. Brummund, M. Kästner, Constitutive modeling of isotropic and anisotropic hyperelastic solids based on physically informed artificial neural networks, 18th European Mechanics of Materials Conference, Oxford, 2022
-
L. Linden, K. A. Kalina, J. Brummund, M. Kästner, An efficient data-driven multiscale scheme based on physics-constrained neural networks and autonomous data mining, 92nd Annual Meeting of the International Association of Applied Mathematics and Mechanics, Aachen, 2022
-
L. Linden, K. A. Kalina, J. Brummund, M. Kästner, An automated data-driven multiscale scheme based on physically informed neural networks, 9th GACM Colloquium on Computational Mechanics 2022, Essen, 2022
Monografien
- L. Linden, Implementierung eines datengetriebenen Algorithmus zur Simulation von Fachwerken mit nicht linear elastischem Materialverhalten, Bachelorarbeit Mathematik, 2022
- L. Linden, Datengetriebene Modellierung anisotroper Elastizität bei finiten Deformationen mittels künstlicher neuronaler Netze, Diplomarbeit Maschinenbau, 2020