High Performance Deep Learning Framework
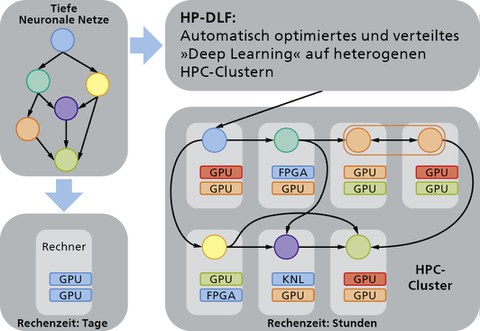
HPDLF Schema
With Deep Learning (DL), machine learning has experienced a rapid upswing in recent years. Image and speech processing or autonomous driving have taken on new dimensions and meanwhile influence our everyday lives.
Science is also increasingly using DL for data analysis. DL is based on processing large amounts of data, especially when learning. Due to the complexity of the algorithms, parallel processing is difficult - the learning process can often take days or even weeks.
Therefore, the BMBF project "High Performance Deep Learning Framework" (HP-DLF) aims to develop methods and a framework for scalable, dynamic and hardware-adapted autoparallelisation. The goal is to provide users with an innovative tool for DL that automatically achieves maximum scalability and energy efficiency on a wide variety of parallel architectures. It is based on existing DL libraries and the Fraunhofer ITWM's GPI-Space autoparallelisation tool.
The main focus of ZIH is on performance and data modeling for the determination of validity statements and performance estimates. However, other factors such as energy consumption should also be taken into account. The models will incorporate both static (compute, memory, network) and dynamic information.
Partners
- German Research Center for Artificial Intelligence
- Fraunhofer ITWM, Competence Center High Performance Computing
- University Heidelberg, Visual Learning Lab
Contact
Andreas Knüpfer
Duration
11/2017-10/2020