Sep 30, 2024
Promotion Hubert Naraniecki
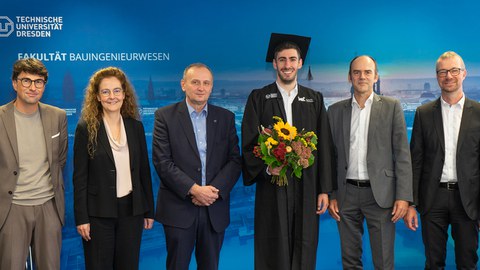
Hubert Naraniecki and the doctoral committee present
On 27.09.2024, Mr. Hubert Naraniecki, M.Sc. successfully defended his scientific thesis as part of the doctoral procedure with the topic "Condition prognosis of railroad bridges using machine learning methods". In addition to the chairman of the doctoral committee, Prof. Dr. Karsten Menzel, (TU Dresden), the reviewers were Prof. Dr. Steffen Marx (TU Dresden), Prof. Dr. Thomas Braml (Universität der Bundeswehr München) and Prof. Dr. Danièle Waldmann-Diederich (Technische Universität Darmstadt). Prof. Dr. Jens Otto (TU Dresden) was also present as a member of the doctoral committee.
Abstract:
The importance of bridges for mobility and the transportation of goods, the advanced age of structures and the increased impact of traffic mean that structural maintenance is becoming increasingly important. For both railroad and road bridges, a reactive maintenance strategy is applied nationally, whereby maintenance measures can only be planned after damage has occurred. The maintenance strategy used leads, among other things, to unpredictable and lengthy restrictions on the availability of the structures. In order to counter the problems described, the current maintenance strategy needs to be changed. The predictive maintenance strategy aims to predict changes in condition with the help of condition prediction models and thus identify and solve problems at an early stage. Among other things, condition prediction models can be based on infrastructure data stored in bridge management systems of infrastructure operators.
This scientific paper deals with condition prediction models for railroad bridges in Germany and their possible application in a maintenance management system. By combining different types of infrastructure data from the bridge management system of DB Netz AG, a data basis for the creation of condition prognosis models was created. Damage was integrated as an essential, previously unused input feature and it was investigated whether this could improve the performance of forecast models. Decision trees as an explainable machine learning method and multi-layer perceptrons as so-called black box models were used for the training of condition prediction models. For the main construction types (reinforced concrete, prestressed concrete, steel, rolled girders in concrete and vaults) of railroad bridges, models for condition prediction were created for the superstructures, abutments and piers. By including damage as a feature, similarly good prediction performance was achieved with both machine learning methods. Furthermore, the most important features of the models were used to show that damage has a significant influence on the condition prediction. The similar prediction performance between decision trees and multi-layer perceptrons showed that simple explainable learning methods can also be used for state prediction based on infrastructure data with damage as a feature. The trained models were evaluated in terms of their practical applicability. For bridges made of prestressed concrete and steel as well as for rolled girders in concrete bridges, balanced models could be trained, which allow a good prediction of all conditions and have a low critical misclassification rate.
At the end of the thesis, the application of the condition prediction models and the associated limitations were demonstrated. The condition prediction models based on damage are dependent on the damage recorded in inspections and the development of the damage characteristics. For a comparison of the predicted conditions, ageing curves based on the type of construction were derived from inspection data with a recording period of approximately 21 years. The ageing curves derived from the condition prognosis models provide a predictive approach for maintenance management and thus a possible basis for changing the maintenance strategy.
We would like to congratulate Hubert Naraniecki on completing his doctorate and wish him all the best and every success in his future endeavors.