Data-based tool commissioning in sheet metal forming
Table of contents
Project duration: |
04/2023 – 03/2026 |
Funding: | Funded by the German Research Foundation DFG as part of the priority program 2422 "Data-driven process modeling in forming technology" |
Project manager: | Dipl.-Ing. Adili Yiming |
Partner: |
Prof. Dr. Oliver Niggemann |
Motivation
Tool commissioning is manual, time-consuming and physically demanding work. It is experience-based and requires an individual approach for each tool. Workshop personnel must process a large amount of heterogeneous data and abstract information and also take into account interactions with subsequent processes. In view of the existing and worsening shortage of skilled workers and the high proportionate costs of tool training in the development process, science must create the basis for its automation.
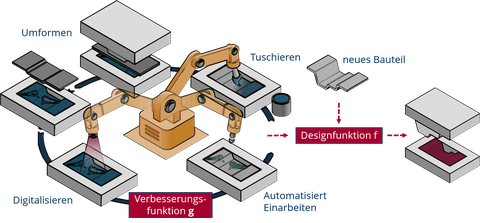
Automated tool commissioning
Objective of the project
Successful tool commissioing in sheet metal forming is a complex task due to the prevailing interactions and high uncertainties. Essentially, two types of degrees of freedom can be influenced: (i) the nature of the active surfaces of the forming tool and (ii) the adjustable parameters of the tool-machine system. In this project, machine learning methods are being developed that can both automate tool commissioning and adapt the design of the active tool surface to the complex interactions of the real system during the tool development process.
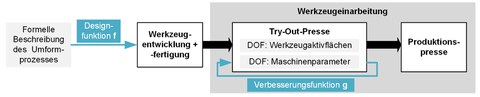
Optimization problem in tool commissioning
The following research questions must be answered in order to learn the improvement function g (corresponds to tool try-out) and the design function f (corresponds to tool design) from data:
Research question 1: How can the human operator be supported in the task of tool try-out at the tryout press or how can this activity be automated? I.e. can we use data and technical know-how to calculate the improvement function and thus automate the activity?
Research question 2: Which type of artificial neural network (ANN) with which network topology is suitable for the automated generation of the active surfaces of forming tools, taking into account the tool-machine interaction?
Research question 3: Which type and topology of ANN is suitable for extending the design function with regard to the dependencies of the active tool surfaces on machine parameters such as force and speed progression?
Approach
Hypothesis 1: A camera-based system for the automated acquisition and analysis of spotting images of the active surfaces and 2D images of the formed component is suitable for determining the necessary removal rate. Optimized machine and process parameters can be calculated from historical data and simulations. Experience from learning the improvement function and experience from the tryout process can be used to improve the design function.
Hypothesis 2: Neural networks that are able to process spatial data can solve the design problem. Any solution must be robust and, above all, enable the integration of a priori knowledge such as physical laws and technical standards. Methods from the field of representation learning or the integration of symbolic AI algorithms could represent possible solutions here. Pre-training with simulations is a possible solution to increase the robustness of ML models despite small amounts of data.
Hypothesis 3: The solution to the problem requires other types of ANN than research question 2, as both scalar values and sequences must be predicted. The design function identifies parameters from descriptions of the forming problem and the machine. A formal description must be defined for both. In this case, the pre-training of ANN on simulation data could also be a solution.
Challenges
- The central challenge is the large number of interacting influencing variables on the quality of the digitized spotting image and its interpretation with regard to the necessary material removal.
- Consideration and optimization of the material flow into the die
- Transfer of the trained ANN to the next tool generation
- Automation of the individual work steps
Path to solution
A comprehensive experimental platform is being set up to provide the database for the development of the ANN and its training, testing and validation phase. The platform provides both real and simulated data and should enable the mapping of different tool configurations. At the same time, the forming process, sensor data and expert knowledge are formalized. Finally, various suitable ANNs are used to calculate the necessary material removal on the active surface of an existing tool and to improve the forecast for a new tool.
Results
- Test set-up for the automatic generation of training data for ANN for the prediction of material removal quantities on the basis of spotting images
- Robot cell for automated tool incorporation including color application to the blank, recording of the spotting pattern and mechanical correction of the active tool surfaces.
Current status
In a first step, camera images and profilometer images of different spotting ink applications on a test specimen (left) were evaluated with a trained artificial neural network. The simple neural network can predict the height of the paint layer (center). The reference height profile image of the profilometer for the same paint application (right) shows a promising agreement with the calculation of the ANN.
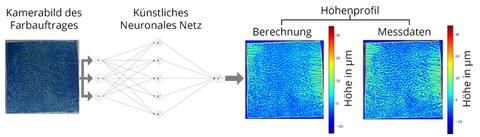
Calculation of the height profile of a spotted surface using KNN
Contact
wissenschaftlicher Mitarbeiter
NameMr Adili Yiming M.Sc.
Maschinenverhalten
Send encrypted email via the SecureMail portal (for TUD external users only).
Chair of Machine Tools Development and Adaptive Controls
Visiting address:
Kutzbach-Bau, Raum E5 Helmholtzstraße 7a
01069 Dresden