Real-Time Capable, Integrated Airline Control System Supported by Machine Learning
Project information
- Funded by DFG
- Duration of 3 years, started in March 2024
In the busy world of air traffic management, delay minimization and operational efficiency are paramount. This project focuses on the development of a machine learning-supported integrated airline control system designed to address and mitigate airline disruptions and delays at their core. The project is executed by two doctoral research associates, each specializing in distinct yet complementary areas of the airline operational spectrum.
Problem Setting
The aviation industry faces significant challenges with turnaround delays and their cascading effects throughout the air traffic management (ATM) network. These delays not only disrupt airline operations but also result in increased costs and reduced passenger satisfaction. Traditionally, these problems have been tackled through separate, often isolated approaches focusing on different operational components such as aircraft, crew, and passenger management. The goal of this project is to improve air traffic control systems by addressing disruptions and delays more effectively using real-time capable, integrated airline control system supported by machine learning. We seek to extend the existing approach of managing turnaround times and traffic flow to involve multiple stakeholders like airports, air traffic control, and traffic flow managers.
Role of Optimization
In the optimization part, technical-analytical tasks in the field of operations research as well as for the implementation of control processes in fleet circulation and ground handling are processed. The focus is on the following optimization tasks:
- Preparation of Operational Correlations: Preparing operational correlations to identify patterns and relationships within the airline operations data. By analysing these correlations, we intend to optimize fleet circulation and ground handling processes to improve efficiency and reduce delays.
- Design and Implementation of Cross-Stakeholder Control Process: Designing and implementing a cross-stakeholder control process for fleet circulation and ground handling. This involves coordinating and optimizing the interactions between different stakeholders involved in the airline operations to streamline processes and enhance overall performance.
- Integration of Control Measures: Integrating control measures derived from data analysis and operational correlations into the optimization framework. By incorporating these control measures, the aim is to enhance decision-making processes and improve the real-time capabilities of the airline control system.
- Flexibilization and Adaptation of Constraints: Flexibilizing and adapting constraints resulting from machine learning predictors. This involves adjusting constraints based on real-time data and insights to improve the efficiency of the control system and optimize decision-making.
Role of Machine Learning
Machine learning (ML) is the second central component of our project and assists in analyzing large amounts of operational data to predict delays and make the airline control system faster and more efficient in dealing with potential disruptions. The focus is on the development and integration of machine learning techniques in this project with the following tasks:
-
Development of Predictive Models: Designing and training machine learning models that forecast reactionary delays and optimize response strategies. These models leverage historical data to discern patterns and predict outcomes under varied operational scenarios.
-
Real-Time Decision Making: Implement machine learning algorithms that support swift and effective decision-making in real time, ensuring that the integrated airline control system can promptly adjust to operational changes and minimize disruption impacts.
-
Collaborative Integration: In partnership with my colleague, who leads the airline operational research, we work together to make sure that the machine learning results fit well into the airline's operations. This teamwork is important to create a solution that is both technically strong and practical for the airline.
Project Impact
The project aims to enhance operational efficiency in the airline control process through an integrated approach and the application of advanced machine learning techniques. The primary focus is on reducing delays and optimizing resource management. This, in turn, is designed to strengthen the operational resilience and adaptability of the airline, ultimately leading to improved passenger service quality and cost reductions associated with delays in the long term.
Contact
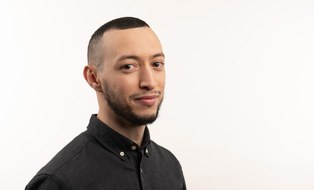
Research Associate
NameAyoub Bouayaben M.Sc.
Send encrypted email via the SecureMail portal (for TUD external users only).
Chair of Air Transport Technology and Logistics
Visiting address:
Gerhart-Potthoff-Bau (POT), Room 361 a Hettnerstraße 1-3
01069 Dresden