ZIH-Kolloquien 2019/2020
23. Januar 2020: Markus Bär (Physikalisch-Technische Bundesanstalt, Berlin)
"Emergence and control of patterns in active fluids"
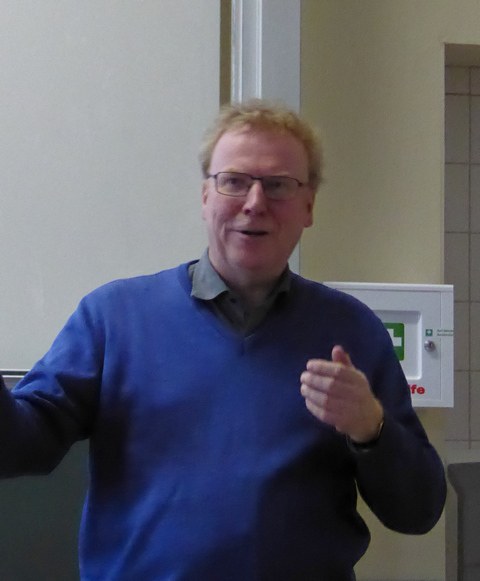
Markus Bär
Active fluids like suspensions of bacteria, microtubule bundles and artificial microswimmers consist of individuals that are able to transform internal energy into a directed motion. The talk surveys a variety of surprising examples for spatiotemporal self-organisation of active matter like mechano-chemical waves in giant amoeba cells and mesoscale turbulence in suspension of swimmers. Many efforts have been made to understand the influence of individual’s properties onto the emergence of pattern on the meso- or macroscale. In the talk, we first formulate “microscopic” equations of motion for a collection of active particles that interact through short-range alignment and long-range hydrodynamic interactions and are subject to rotational and translational noise. A derivation of “mesoscale” continuum equations from the equations of motion of this collection of interacting model swimmers will then be sketched. Depending on the symmetry of the interaction between swimmers the continuum equations obtained lead to polar or nematic order parameter equations coupled to the hydrodynamic equation of the surrounding fluid. As a result, the different symmetries lead to the emergence of different topological defect as well as different forms of collective motion and patterns. Here, the phenomenon of mesoscale turbulence is discussed in detail and compared to recent experiments. Finally, the topic of control of emerging patterns in swimmer suspensions by means of external fields and periodic arrays of obstacles is addressed.
13. Dezember 2019, 14:00, Andreas-Pfitzmann-Bau APB 1096:
Marek Kimmel (Rice University, Houston and Silesian University of Technology)
"Statistical Inference of growth and mutation patterns of tumors based on genomic data"
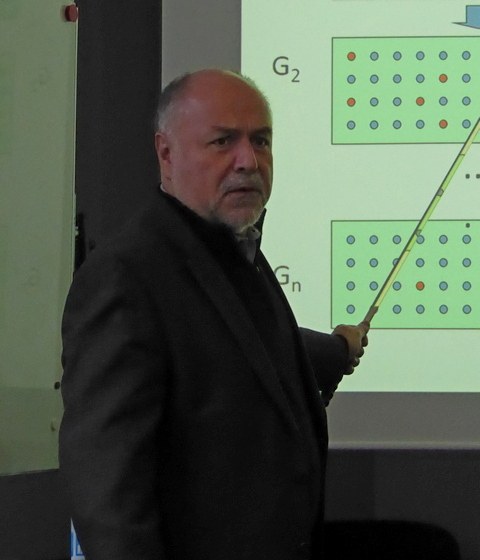
Marek Kimmel
We present a mathematical model of growth of secondary clones in cancer cell populations, anchored in the coalescence theory. Recent years have seen considerable work on inference about cancer evolution from mutations identified in cancer samples. Much of the modeling work has been based on classical models of population genetics, generalized to accommodate time-varying cell population size. Reverse-time, genealogical views of such models, have been used to infer aspects of the past of growing populations. Another approach is to use branching processes, the simplest scenario being the classical linear birth-death process. Inference from evolutionary models of DNA often exploits summary statistics of the sequence data, a common one being the so-called Site Frequency Spectrum (SFS). In a bulk tumor sequencing experiment we can estimate for each site at which a novel somatic point mutation has arisen, the proportion of cells that carry that mutation. These numbers are then grouped into collections of sites which have similar mutant fractions. We examine how the SFS based on birth-death processes differs from those based on the coalescent model. This may stem from the different sampling mechanisms in the two approaches. However, we also show that despite this, they are quantitatively comparable for the range of parameters typical for tumor cell populations. We also present a model of tumor evolution with selective sweeps, and demonstrate how it may help in understanding the history of a tumor as well as the influence of data pre-processing. Based on the model, we describe difficulties in reconstructing past demography of tumors. Considerations are illustrated by analysis of site frequency spectra of tumors from The Cancer Genome Atlas.
5. Dezember 2019, 15:00, Willers-Bau A 317:
Alberto Saldaña (Institute of Mathematics, National Autonomous University of Mexico, Mexiko-City)
"Asymptotic partial symmetries to reaction-diffusion systems coming from ecology"
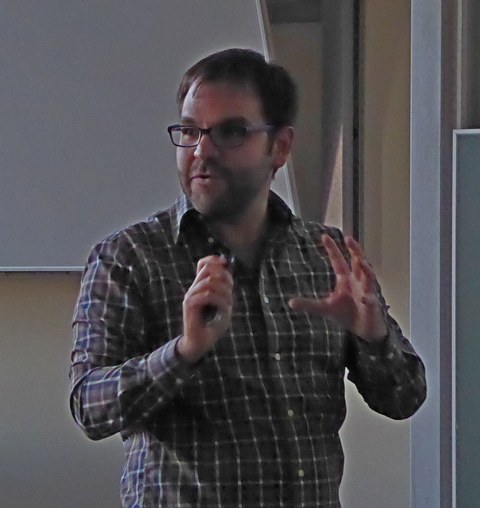
Alberto Saldaña
The interaction between two species can be modeled by a system of two nonlinear reaction-diffusion equations. The equation takes into account the movement of the species, the birth rates, the consequences of concentration, and the interaction between the two populations, which can be cooperative or competitive. These models are sometimes called of Lotka-Volterra type and are complemented with boundary conditions and an initial profile. In this talk, I focus on the shape of solutions for large times; in particular, I show that, under certain assumptions, the solutions exhibit a phenomenon called "asymptotic symmetry", that is, all the elements in the omega-limit set have some kind of symmetry (which depends on the hypothesis), as well as some extra monotonicity properties.
Alberto Saldaña studied Actuarial Sciences in Mexico City at the National Autonomous University of Mexico (UNAM), where he also did his M.S. in Mathematics. He obtained his Ph.D. in Mathematics from the Johann-Wolfgang Goethe-Universität Frankfurt am Main, where he studied qualitative properties of solutions to reaction-diffusion equations. Alberto has done three postdoctoral stays: the first at the Université libre de Bruxelles ( ULB ) in Belgium, where he studied differential equations of higher-order which model phase separation; the second stay was at the Instituto Superior Técnico ( IST ) in Portugal, where he studied nonlinear elliptic systems. His third postdoc, with the support of the Alexander von Humboldt Stiftung, was at the Karlsruhe Institut für Technologie ( KIT ), where his research was focused on the study of quasilinear differential operators used in models of electromagnetism. Also during this period he studied pseudodifferential operators which model processes where the diffusion has jumps.
4. Dezember 2019, 13:00, Trefftz-Bau, TRE 101:
Yanko Davila, Michael Mueller, Alfredo Herrera (Cognitec Systems, Dresden)
"Ceph: Experiences and Lessons Learned @ Cognitec"
Storing your organization's data represents a big challenge. Choosing a solution
often involves a compromise between reliability, scalability, disaster recovery
and operating costs. Ceph is a free software project that offers a solution for an
object storage cluster running on commodity hardware. Ceph’s CRUSH
algorithm gives it an advantage in scalability and performance when compared
to solutions requiring centralized data table mapping. Data is automatically
replicated and rebalanced within the cluster. This level of automation makes
disaster recovery simpler and helps in reducing operating costs. The
presentation aims to share the experiences and lessons learned in the process
of adopting Ceph as our main storage platform as well as integrating it as part
of our products.
28. November 2019, 10:00, Willers-Bau A317: Estela Suarez (FZ Jülich)
"The Modular Supercomputing Architecture: hardware composability for application diversity" (Folien)
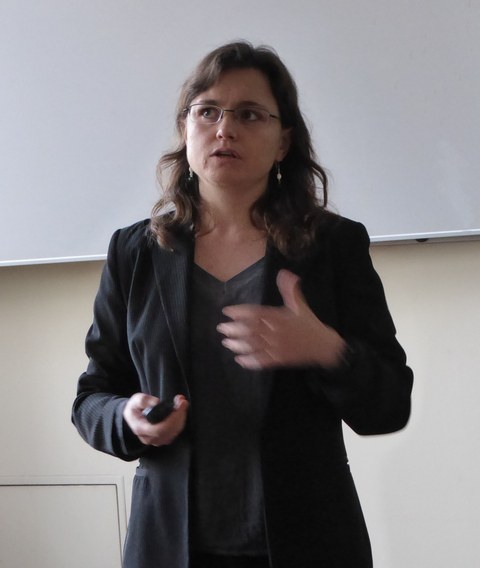
Estela Suarez
The way supercomputers have been built across history has evolved driven by the constant need to increase performance at an affordable cost. Recently the trend goes towards heterogeneous configurations combining general purpose processing units (CPUs) with acceleration devices (e.g. graphic cards (GPUs) or many-core processors). The Modular Supercomputing Architecture developed at the Jülich Supercomputing Centre implements heterogeneity at system-level by segregating CPU and acceleration resources, and strives to provide cost-effective computing at extreme scale fitting the needs of a wide range of Computational Sciences. In a modular supercomputer each application can dynamically decide which kinds and how many nodes to use, mapping its intrinsic requirements and concurrency patterns onto the hardware. Codes that perform multi-physics or multi-scale simulations can run across compute modules thanks to a global system-software and programming environment. Application workflows that execute different actions after (or in parallel) to each other can also be distributed in order to run each workflow-component on the best suited hardware, and exchange data either directly (via message-passing communication) or via the file-system.
This talk will explain the concept of Modular Supercomputing including its background and evolution, how it is built and programmed, and show-case results from scientific applications that are profiting from it.
Dr. Estela Suarez is Senior Scientist and deputy-lead of the Technology Department at the Jülich Supercomputing Centre, which she joined in 2010. Her research focuses on HPC system architectures and codesign. As leader of the DEEP series of EU-funded projects she has driven the development of the Cluster-Booster and the Modular Supercomputing Architectures, including hardware, software and application implementation and validation. Additionally, since 2018 she leads the codesign efforts within the European Processor Initiative. Since 2018 she gives lectures on HPC architectures at the University of Bonn. She holds a Phd in Physics from the University of Geneva and a Master degree in Astrophysics from the University Complutense of Madrid.
2. September 2019, 14:00, APB 1004 (Gr. Ratssaal): James Alexander Glazier (Indiana Univeristy / USA, Biocomplexity Institute)
"Virtual Biological Tissue Simulations and Standards - Opportunities and Challenges"
Current cell atlas projects are generating exceptionally detailed series of snapshots of the molecular states of individual biological cells, and the spatial context of those cells in a variety of normal and abnormal tissues. However, using those atlases to generate biological understanding or to develop therapeutic interventions requires a practical way to generate dynamic virtual-tissue simulations (VTs) from these big data. VTs depend on the ability to take experimental spatial images, populate them with computational model components from repositories and to customize the states and parameters of those components from experimental data.
Unfortunately, we are far from being able to do most of these steps, largely because current computational models do not correspond to what we know about biological modularity or separate the underlying biological concepts and hypotheses from methodological details of implementation. Moreover, current software tools are not designed as interoperable components. As a result, a researcher trying to reuse a computational biology result often needs to try to extract the underlying biological model from incomplete specifications in publications and then translate it into a new mathematical and/or computational instantiation. Based on more than a century of experiences with our popular software CompuCell3D, we outline the success stories as well as difficulties in building and applying VTs and discuss possible areas of progress.
Prof. Dr. Glazier received his B.A. in Physics and Mathematics from Harvard University and his M.S. and Ph.D. in Physics from the University of Chicago. Dr. Glazier’s research focuses on embryonic development, developmental and chronic toxicity and developmental diseases. He leads the collaborative development of the open-source CompuCell3D multi-scale modeling and model-sharing environment. In 2016, he became one of the founding members of Indiana University’s Department of Intelligent Systems Engineering, which aims to apply advanced computing techniques to understand and control complex natural and engineered emergent systems.
25. Juli 2019: Kristian Kersting (TU Darmstadt, Machine Learning Group)
"Deep Machines That Know When They Do Not Know"
Our minds make inferences that appear to go far beyond standard machine learning. Whereas people can learn richer representations and use them for a wider range of learning tasks, machine learning algorithms have been mainly employed in a stand-alone context, constructing a single function from a table of training examples. In this talk, I shall touch upon a view on machine learning, called probabilistic programming, that can help capturing these human learning aspects by combining high-level programming languages and probabilistic machine learning — the high-level language helps reducing the cost of modelling and probabilities help quantifying when a machine does not know something. Since probabilistic inference remains intractable, existing approaches leverage deep learning for inference. Instead of “going down the full neural road,” I shall argue to use sum-product networks, a deep but tractable architecture for probability distributions. This can speed up inference in probabilistic programs, as I shall illustrate for unsupervised science understanding, and even pave the way towards automating density estimation, making machine learning accessible to a broader audience of non-experts.
This talk is based on joint works with many people such as Carsten Binnig, Zoubin Ghahramani, Andreas Koch, Alejandro Molina, Sriraam Natarajan, Robert Peharz, Constantin Rothkopf, Thomas Schneider, Patrick Schramwoski, Xiaoting Shao, Karl Stelzner, Martin Trapp, Isabel Valera, Antonio Vergari, and Fabrizio Ventola
Kristian Kersting is a Professor (W3) for Machine Learning at the Computer Science Department of the TU Darmstadt University, Germany, heading the machine learning lab and being a member of the Centre for Cognitive Science. After receiving his Ph.D. from the University of Freiburg in 2006, he was with the MIT, Fraunhofer IAIS, the University of Bonn, and the TU Dortmund University. His main research interests are statistical relational artificial intelligence (AI) and probabilistic deep learning. Kristian has published over 160 peer-reviewed technical papers and co-authored a book on statistical relational AI. He received the European Association for Artificial Intelligence (EurAI, formerly ECCAI) Dissertation Award 2006 for the best AI dissertation in Europe, a Fraunhofer Attract Research Grant with a budget of 2.5 Million Euro over 5 years (2008-2013) and three best-paper awards (ECML 2006, AIIDE 2015, TPM 2019). Kristian is a Fellow of the European Association of Artificial Intelligence (EurAI) and was named Top 100 Influential Scholar 2018 for Aritificial Intelligence by AMiner. Kristian co-chaired the PC of ECML PKDD 2013 and UAI 2017, and is an elected PC co-chair of ECML PKDD 2020. He is the founding Speciality Co-Editor-in-Chief for Machine Learning and AI of Frontiers in Big Data, and (past) action editor of TPAMI, JAIR, AIJ, DAMI, and MLJ.
10. Juli 2019, 14:00, APB 1004 (Gr. Ratssaal): Debojyoti Chakraborty (CSIR-Institute of Genomics and Integrative Biology
New Delhi, India)
"CRISPR 2.0 : therapeutic genome editing and modelling neuropathies using 'mini brains’"
Clinical trials using CRISPR Cas9 have begun for a number of genetic defects. However, concerns over off-targeting and unwanted DNA breaks have lingered leading to continuous efforts to identify more potent genome editing tools. My lab has identified an orthogonal Cas9 protein with very high degree of specificity in cells owing to fundamentally different DNA interrogation properties. We have used this protein to correct the sickle cell anemia mutation in patient derived iPSCs and have developed an adenine base editor for making double strand break-free changes in DNA. Using these tools, we are involved in using CRISPR-mediated perturbations in healthy and patient derived brain organoids and study developmentally associated changes.
Debojyoti Chakraborty is a senior scientist at the CSIR Institute of Genomics and Integrative Biology, New Delhi, India. After completing his PhD and Postdoctoral studies at the Max Planck Institute of Molecular Cell Biology and Genetics and TU Dresden, he is heading a lab working in the field of developing next generation genome editing technologies for therapeutic correction of genetic disorders. He is an Assistant Professor at Academy of Scientific and Innovative Research and is a recipient of the Inspire, Wellcome Trust and Lady Tata Young Researcher awards. He is also an accomplished musician and performs with the Dresden based musical group ‘Dhun’.
6. Juni 2019: Robert Henschel, Kris Ying (Indiana University), Holger Brunst (TU-Dresden)
"Overview of the Collaboration between ZIH and Indiana University"
For more than 15 years the Center for Information Service and High Performance Computing at Technische Universität Dresden has had an active collaboration with the Research Technologies division of Indiana University, USA. For the past 10 years, this collaboration has been formalized in a memorandum of understanding, covering topics that both institutions work on as well as establishing a framework for exchanging researchers and staff. In this talk, Robert Henschel, Director of Research Software and Solutions and Kris Ying, human resources specialist within the IT human resources department, will present a broad overview of the collaboration. The talk will highlight examples of topics that or of interest to both institutions, mainly in the areas of high performance computing and providing computational and storage services to researchers. A major component of the collaboration is the yearly exchange of staff and researchers between the two organizations and we will present the logistical details of such exchanges and highlight future opportunities for research visits.
23. Mai 2019: Jochen Kursawe (University of Manchester)
“Quantitative approaches to multi-scale modelling of epithelial morphogenesis”
Recent years have seen a rise in quantitative data for many biological applications. These new data can lead to challenges at each stage of the scientific method. We need to design quantitative hypotheses through mathematical models, make quantitative experimental predictions, devise methods for quantitative data analysis, and design methods for quantitative inference using models and data. Here, I will talk about how Chaste, an open-source software library for multi-scale modelling in biology and physiology, can facilitate this quantitative transition for the integrative analysis of morphogenesis in epithelia, one of the major tissue types in animals. At the example of tissue size control in the Drosophila embryonic epidermis I show how quantitative multi-scale models can be designed to make experimentally testable predictions. I further present a novel algorithm for robust cell tracking in live-imaging microscopy videos of epithelial tissues that illustrates how graph theoretic concepts may be used to overcome challenges in quantitative data analysis. Finally, I discuss a Bayesian method for estimating mechanical parameters of cell-based models from imaging
data that allows quantification of parameter uncertainty.
Jochen studied for his Diplom degree in physics at the Technical Universities of Dresden and Munich, and at the University of Alberta, Canada. He conducted his doctoral studies at the Mathematical Biology group at the University of Oxford and is now a postdoc in Nancy Papalopulu's lab at the University of Manchester. Jochen applies quantitative computational approaches to understand multi-scale interactions and timing during embryonic development.
4. April 2019, 15:00, WIL A317:
Konstantinos Xylogiannopoulos
"High performance computing using low performance computers: How to perform advanced pattern detection using minimal resources."
For the past two decades, the big data concept has been invading our lives. A huge variety of electronic devices produce enormous numbers of data every day. A complex and diverse universe of mobile, stationary, wearable and other kinds of devices and sensors floods our computers with vast datasets, defined by the three, five or seven Vs
of big data. However, another parallel, quiet, very beautiful and infinite universe awaits to surprise us with its simplicity and potential in big data science: mathematics. Number theory provides us with the biggest dataset we could ever imagine having access to. With the incredible number of 31.4 trillion decimal digits or 28.57 TB (Emma
Haruka Iwao, 2019), π is the biggest, most intrinsic and fascinating, single piece of information we probably know so far; and it gets bigger. Remove, alter or insert just one digit and we have something completely different. Either we can analyze π as it is, or we cannot. In this lecture we will examine how π, irrational numbers in general, can be used to define the problem of detecting all repeated patterns existing in a string. Novel, problem specific, pattern detection data structures and algorithms will be presented, which allow us to perform amazing results, very fast, using limited resources. We will examine how methodologies for big data analytics, built on top of these data structures and algorithms, can be used in supercomputers to perform instant results by maximum utilization of parallelization. Furthermore, we will investigate the importance of these methodologies and how the outcome of all repeated patterns detection can formulate the basis for solving problems in many other artificial intelligence and data analytics fields, such as text mining, frequent and non-frequent sequential itemsets detection, network security, anomaly detection, bioinformatics, forecasting, recommendation systems, image analysis and many more.
Konstantinos holds a PhD in computer science from the University of Calgary, AB, Canada. He has professional experience in software engineering and financial analysis while currently holds a position of big data scientist in powertrain industry. He specialized in data structures and algorithms for single, multiple and all repeated patterns detection, existing in single, multivariate or multi-dimensional sequences, with simultaneous space and time complexity optimization. He has several publications with applications in many, diverse, fields such as data/text mining, time series analysis, web/network analytics and security, sequential itemsets detection and many more.
7. März 2019, 15:00 Uhr, WIL A317:
Paul Messina (Argonne National Laboratory)
"The High-Performance Computing Ecosystem"
Advances in High-Performance Computing (HPC) are often attributed to improvements in hardware technologies or more recently to the deployment of systems of unprecedented scale. However, advances in simulation and modeling in science and engineering – the reason more powerful computer systems are designed and built – require a broad ecosystem.
Among the components of the ecosystem are analytic and numerical mathematics, mathematical software, software libraries, software tools, data analysis and visualization methods and software, micro and macro architecture, ability to move data internally and externally, and global computer networks. And those components have to be integrated so that they can be used productively and opportunities for further research and improvement can be identified.
Examples from the last four decades will be used to support this view of HPC.
27. Februar 2019, 11:30 Uhr, APB 1096:
Robert John Noble (ETH Zürich)
"Characterising the evolutionary modes of cancer and normal tissue"
The nature of evolution within normal and neoplastic tissue is a subject of debate. I will present a highly flexible computational model that allows evolutionary dynamics resulting from diverse spatial structures to be compared in a single framework. Combining stochastic simulations with mathematical analysis, I will explain how tissue architecture governs the potential for subclonal expansion, the prevalence of selective sweeps, and spatial patterns of genetic heterogeneity. I will describe the conditions under which genetic diversity is most predictive of tumour progression, and I will discuss applications in optimising treatment protocols and understanding cancer risk variation. These findings help explain the observed multiformity of cancer and normal tissue evolution and contribute to establishing a theoretical foundation for predictive oncology.
30. Januar 2019 , 11:00, APB 1096:
Fernando Peruani (University of Nice)
„Intermittent behavior across scales in biology“
Intermittent behavior is observed in biological systems at all scales, from bacterial systems to sheep herds. First, I will discuss how Escherichia coli explores surfaces by alternating stop and moving phases. Specifically, I will show that a stochastic three behavioral state model is consistent with the empirical data. The model reveals that the stop frequency of bacteria is tuned at the optimal value that maximizes the diffusion coefficient. These results provide a new perspective on how evolution may have reshaped bacterial motility apparatus. Intermittent motion is also observed in sheep, where again a stochastic three behavioral state model provides a quantitative understanding of the empirical data. However, in sheep, individual transition rates depend on the behavioral state of other individuals and collective behaviors emerge. Specifically, I will show that small sheep herds display highly synchronized intermittent collective motion, with the herd behaving as a self-excitable system.