Poetsch Gruppe
Die Poetsch-Gruppe setzt Computertechniken und Ansätze des maschinellen Lernens ein, um DNA-Schäden und Reparaturprozesse, Mutagenese und Genombearbeitung zu bewerten und zu modellieren, um klinische Anwendungen zu entwickeln. Die Gruppe etablierte eine neuartige Technik für genomweite Messungen von oxidativen DNA-Schäden und trug zum Verständnis der Präzision der Genombearbeitung bei. Es wird daran gearbeitet, dies im Zusammenhang mit verschiedenen Krebsarten und als Folge unterschiedlicher Behandlungsschemata gewebespezifisch zu verstehen.
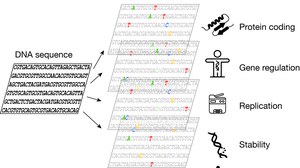
Unsere Forschung
The Biomedical Genomics group employs computational techniques, machine learning, and deep learning approaches to assess the layers of information genomes encode. In this context we are particularly interested how the genome functions in the context of balancing function with DNA damage and mutagenesis.
The group established a novel technique for genome-wide measurements of oxidative DNA damage and contributed to the understanding of genome editing precision and the genome wide distribution of mutations.
For this we use computational biology with different types of functional genomics data (AP-Seq, HiC, ChIP-Seq,...) and cancer genomics data and different machine learning and deep learning techniques, particularly the DNA language models we develop, GROVER and EAGLE-MUT.