Sep 26, 2017
Researchers of TU Dresden have pioneered a brain-network bio-inspired algorithm to predict new therapeutic targets of approved drugs
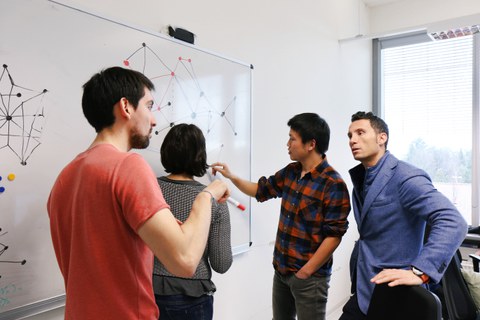
Members of Dr. Carlo Vittorio Cannistraci’s research group
An international team of scientists led by Dr. Carlo Vittorio Cannistraci, Junior Group Leader of the Biomedical Cybernetics lab at the BIOTEChnology Center TU Dresden, has developed a powerful computational method that can exploit the principles of brain-network self-organization to predict new, highly reliable drug-target interactions using only the topology of molecular networks without any further information about the chemical structures of the drugs.
<<The brain is just the weight of God, […] >> with these words Emily Dickinson described the magnificence of the brain. However, even for a creative mind, it would be difficult to prefigure that some brain-network wiring rules follow generalized principles of complex system organization to such an extent that those principles can be used to predict interactions between known drugs and new therapeutic targets in molecular networks.
The cost of drug development is currently estimated to be more than $1 billion US for every new drug that enters the market and that cost is constantly increasing. Drug repositioning, which aims to identify new applications for approved drugs, is a promising solution to this economic problem. However, drug repositioning requires new potential targets for known (approved) drugs to be identified. For this reason, a plethora of methods and data sets have been proposed and tested to address the problem of drug–target interaction prediction.
Two peculiarities make special the drug–target interaction prediction method proposed by Dr. Cannistraci and colleagues. The first is that it exploits a self-organization rule derived from brain networks to predict new drug–target connections. The second is that it can predict new drug-target interactions by exclusively using a known network of previously established interactions, without additional biological or molecular knowledge, such as the chemical similarity of drugs and the protein sequence similarity of targets. The ability to predict new interactions emerges directly from the nonlinear structural organization of a complex network. On the basis of this observation, some years ago Dr. Cannistraci developed a theory entitled Local-Community-Paradigm (LCP). According to LCP, several real complex networks have a structural organization consisting of many local communities that, similarly to the brain, favour local signalling activity, which in turn promotes the development of new connections within those local communities. This idea was inspired by the famous assumption behind Hebbian learning, a hypothesis proposed by the psychologist Donald Olding Hebb during the late 40s: neurons that fire together wire together. Dr. Cannistraci noticed that the network topology plays a crucial role in isolating cohorts of neurons in functional modules that can naturally and preferentially perform local processing because the local-community organization of the network topology creates a physical and structural ‘energy barrier’ (a topological gap between distinct communities) that confines these neurons to preferentially fire together within a certain community and consequently to create new links within that community. This process implements a type of local topological learning that Dr. Cannistraci has termed epitopological learning, which stems as a general topological interpretation of Hebbian learning, whose definition was only given for neuronal networks. Hence, epitopological learning and the associated local-community-paradigm (LCP) have been proposed as local rules of learning and organization, which are generally valid for modelling link-growth and for topological link prediction in any complex network with LCP architecture. In this new study published in Briefing in Bioinformatics – one of the leading journals of the field - the LCP theory and the derived algorithms for link prediction based on epitopological learning proved to give an outstanding performance also in network-based drug-target prediction.
“I was amazed when I obtained the first results of our study. They showed that a simple and unsupervised rule of organization derived from the brain network could perform much faster and offered comparable prediction performance to that of complicated algorithms based on advanced, supervised and knowledge-based engineering. In the future I would like to focus on developing new hybrid and adaptive algorithms that merge the benefits of both the strategies of prediction”, explains Claudio Durán, one of the first authors of the study.
“The brain network and the rules behind its self-organization fascinated me since I was in high school. I decided to dedicate my life to study how the organization of the brain network functions to represent and predict the world around us. In our study, we show that some of the simple rules that apply to brain network organization (to implement its learning ability) are also general rules of self-organization of complex networks. For such reason, these rules can be applied to successfully predict, in a matter of seconds, the interactions of molecular networks without any knowledge of the molecular characteristics, but instead by only exploiting the way the network is organized. The conclusion is that many systems could perform very fast learning by modifying their structure, since their connectivity is already organized to indicate where structural modifications should emerge. This principle can be used to design new efficient algorithms for link-prediction in many types of networks in general“, says Carlo Vittorio Cannistraci, the corresponding author of the study.
The study was supported by the Klaus Tschira Stiftung GmbH (KTS) foundation and was conducted in collaboration with the Bioinformatics group at BIOTEC led by Prof. Michael Schroeder.
Carlo Vittorio Cannistraci is a Theoretical Engineer. He has been Junior Group Leader of the Biomedical Cybernetics lab at BIOTEC since February 2014 and he is a Young Investigator of the Department of Physics at TU-Dresden since 2016. His research interests include studying the interface between the physics of complex systems, complex networks and machine learning theory.
Claudio Durán is currently undertaking his PhD in the junior group of Dr. Carlo Cannistraci. He is studying machine learning and network biology techniques for exploration and analysis of omics data in systems biomedicine.
Publication
“Pioneering topological methods for network-based drug-target prediction by exploiting a brain-network self-organization theory”
Claudio Durán*, Simone Daminelli*, Josephine M. Thomas*, V. Joachim Haupt, Michael Schroeder & Carlo Vittorio Cannistraci
*contributed equally
Briefings in Bioinformatics, bbx041 (2017)
DOI: 10.1093/bib/bbx041
https://academic.oup.com/bib/article-lookup/doi/10.1093/bib/bbx041
Media inquieries:
Franziska Clauß, M.A.
Press Officer
Tel.: +49 (0) 351 458 82065
The Biotechnology Center was founded in 2000 as a central scientific unit of the TU Dresden with the goal of combining modern approaches in molecular and cell biology with the traditionally strong engineering in Dresden. The BIOTEC is fostering developments in the field of Biotechnology and focuses on cell biology, biophysics and bioinformatics. Since 2016 the Biotechnology Center is part of the central scientific unit “Center for Molecular and Cellular Bioengineering” (CMCB) of the TU Dresden. https://www.biotec.tu-dresden.de https://www.tu-dresden.de/cmcb