00101010? Everything digital? ... Everything digital!
Is 42 the answer to all questions? Or is it digitalization? We are not sure either. But we are convinced of the power of digitization, which leads to faster development of industrial processes, resource-saving procedures, and higher-quality jobs by eliminating routine work.
That is why, for example, work is being done on digitally networked laboratories at the Chair of Bioprocess Engineering in the SmartLab Systems research group. This includes the development of standards for communication between laboratory devices and the robotization and automation of work processes in the laboratory through design, electronics development, and programming. The smart lab of the day after tomorrow must also be flexible and easy to operate while recording all process data. For this purpose, there is the iHEX system in the laboratory of the professorship as an experimental field for laboratory digitalization from collaborative robots without disturbing enclosure (cobot) to assisted workflow.
In addition, we are picking up on the trend towards ever greater miniaturization and shrinking our sensors until they float freely as small "Sens-O-Spheres" in bioreactors and wirelessly transmit measured values to the process controllers. By working on these topics, our students are well versed in the Internet of Things (IoT) and the project planning and development of digitized device solutions and work processes with CAD, microcontrollers, and rapid prototyping (3D printing).
Artificial intelligence - learning from nature
Engineers use various software tools to dimension process engineering apparatus and develop processes based on them. These tools contain mathematical models that can be used, for example, to predict the amount of material converted in the apparatus as a function of temperature and pressure.
In addition to classical process models, which are based on conservation laws for mass, energy, and momentum and combine various chemical, thermodynamic and physical laws, data-driven models such as neural networks, which are based on the thought processes in human brains and are trained using large amounts of data, are increasingly being used.
At the Chair of Chemical Process and Plant Engineering, we develop advanced classical process models and explore how neural networks can be used to model chemical reactors more precisely and quickly.
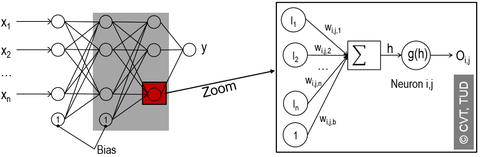
Schematische Struktur eines neuronalen Netzes zur Vorhersage des Prozessparameters y auf Basis verschiedener Eingangsinformationen x1…xn.
Digitalization and artificial intelligence in the process industry
Like many other industries, the process industry expects significant advances from digitalization and artificial intelligence. The application of digital methods, technologies, and artificial intelligence in the process industry is diverse. For example, we can make the planning of process plants much more resource- and energy-efficient based on information models, simulation models, and with the help of artificial intelligence. The same methods and technologies also help us optimize plant operation. Knowledge-based and data-driven models support the monitoring of plant operation and the diagnosis of operational faults and allow efficient planning of maintenance measures. In addition, plants can be automatically optimally controlled and regulated with the help of algorithms. Here, too, the aim of optimization is a resource- and energy-efficient operation and an increase in economic efficiency and product quality.
Crucial to the success of digital methods and technologies is the quality of the digital representation of knowledge about real systems such as the overall plant, individual apparatuses (e.g., reactors or columns), the processes taking place (e.g. of water electrolysis), and the material system in machine-readable form.
In the Systems Process Engineering group, we research methods for mapping physical and chemical relationships in the form of mathematical equations as well as their implementation in the form of efficient algorithms and for simulation and optimization. Furthermore, we combine this behavior knowledge with structural and topological information in knowledge graphs. Moreover, we work on integrating this information and methods with machine learning and computer vision methods to generate valuable information from experimental and operational data.
Modular process plants
The process industry must constantly adapt to new conditions and face new challenges. The loss of raw materials due to disrupted supply chains or fluctuating demand for specialized products are now part of everyday life. Conventional, large process plants that, for example, already take days to start up and shut down cannot meet these challenges. That's why we need to make production plants more flexible. The solution to this problem is the modularization of process plants.
Suppose process plants are designed from individual modules for feeders. In that case, reactors, filters, etc., can be freely combined, and the planning and engineering of process plants are transformed into a simple selection of the appropriate process units for the desired plant. Furthermore, modules can be integrated across manufacturers by standardizing physical and information technology interfaces. This offers new flexibility in the process plants' planning, construction, integration, and operation.
In their joint research, the Systems Process Engineering group and the Chair of Process Control Engineering deal with the design and automation of modules. They also develop methods and software for rapidly planning and integrating modular plants.