Apr 13, 2017
Dresden researchers have developed an intelligent algorithm that automatically identifies significant associations between latent variables in big data sets
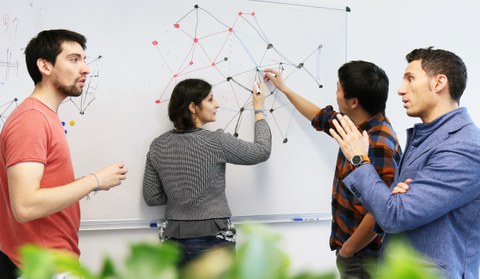
Research group of Dr. Carlo Vittorio Cannistraci
An international team of scientists led by Dr. Carlo Vittorio Cannistraci, group leader of the Biomedical Cybernetics lab at the BIOTEChnology Center TU Dresden, developed ‘PC-corr’: an intelligent algorithm that can automatically discover key groups of interacting latent variables generating differences in big data. PC-corr has detected important molecular signatures in more than six different fields of omic science (e.g. lipidomics, metagenomics, genomics and mechanomics), a step forward towards combinatorial biomarker discovery in precision medicine.
Algorithms are self-contained sequences of instructions devised to solve a certain problem. At the foundation of the current computing revolution, there is a particular class of algorithms called ‘Intelligent Algorithms’, which are able to replicate advanced human abilities that require intelligent behaviour. For example, the ability to look at a set of clinical variables and understand which associations between them lead to a robust diagnosis of pathology, is characteristic of a very well trained and experienced medical doctor. In brief, the ‘intelligent behaviour’ of a doctor is not to consider single variables that deviate from a normal control value individually, but to empower the diagnosis by looking for associations between combinations of discriminative variables that vary together. This type of intelligent reasoning is replicated by ‘PC-corr’, an algorithm invented by Dr. Cannistraci, realized by the Biomedical Cybernetics Group and tested in collaboration with a team of international scientists specialized in more than six different fields of omic science - such as lipidomics, metagenomics, genomics and mechanomics. The study was supported by the Klaus Tschira Stiftung gGmbH (KTS) foundation and involved collaborations with academic partners in Germany such as the Cellular Machines group at BIOTEC, the Center for Regenerative Therapies TU Dresden (CRTD), Andrej Shevchenko group at the Max Planck Institute of Molecular Cell Biology and Genetics (MPI-CBG); as well as abroad such as the Department of Stem Cell Biology at the University of Nottingham (UK) and the Integrin Signalling Group at Fundación Centro Nacional de Investigaciones Cardiovasculares Carlos III (CNIC) in Madrid (Spain). Importantly, Lipotype GmbH, an industrial partner and expert in Lipidomics for health, was involved. The study also received data support from the RIKEN Omics Science Center (OSC) in Yokohama (Japan) and the FANTOM consortium.
PC-corr, when applied to extremely large data sets containing many variables (big data), uses unsupervised machine learning analysis to automatically discriminate cohorts of samples based on different trends in the multidimensional variable space. In addition, PC-corr pinpoints how the variables (e.g. levels of different lipids in the blood plasma) cooperate and self-organize together into sub-network modules that underlie the sample/patient discrimination. Unlike previous algorithms developed for biomedicine that focus only on genomic analyses, PC-corr is a general intelligent algorithm that can be applied to any kind of dataset, including diverse molecular big datasets, and it can highlight the combinations of factors that explain the biological differences between the samples/patients. In particular, the algorithm was tested on big data generated from different areas of omic science, which includes all the fields of molecular biology that generate a characterization of a biological system as a large ensemble of homogenous molecular features. For instance, lipidomics is the study of all cellular lipids in a biological system, while proteomics is the study of all the proteins in a biological system.
The input for PC-corr is a big dataset that contains a large number of variables and the output is a visual network of the significant connections that underlie the biological differences between samples. For example, when a large genomic biomedical data set is analysed, PC-corr squeezes out from thousands of genes a reduced genetic signature that can then be used to design combinatorial and multiscale biomarkers (see picture 2). Biomarkers in the field of biomedicine refer to any measurable biological characteristic, like molecules or clinical variables, which can be used as an indicator of a biological state, condition or process. They can be used in basic and clinical research to provide prognostic information or to monitor the effect of a drug treatment.
“In one of the analysed datasets, the subnetworks of genetic variations spotted by PC-corr were able to explain the major genetic differences between two Japanese sub-populations (one from Tokyo and the other from Okinawa), providing novel combinatorial associations between disease-risk-related variants that occur in Tokyoites”, explains Sara Ciucci, the first author of the study.
“Our algorithm has the unique feature that it can combine variables of the same biological system even though they are measured using difference scales. For instance, in the Mechanomic study we combined genomic and mechanic variables of cancer stem cells derived from patients. In the next months, we want to further develop this algorithm. Our long-term aim is to develop an Artificial Intelligence (AI) ‘scientific assistant’ that can interact directly with the researchers, analyse data automatically (without human programming) and provide automatic combinatorial and multiscale biomarker design“, says Carlo Vittorio Cannistraci, the corresponding author of the study.
Carlo Vittorio Cannistraci is a Theoretical Engineer. He has been the Group Leader of the Biomedical Cybernetics group at BIOTEC since February 2014 and he is a TUD Young Investigator of the Department of Physics at TU Dresden since 2016. His research interests include studying the interface between the physics of complex systems, complex networks and machine learning theory. From 2010 to 2013, he worked as a Researcher and Postdoc at the King Abdullah University of Science and Technology (KAUST, Jeddah, Saudi Arabia) and the University of California at San Diego (UCSD). Carlo Cannistraci obtained his doctoral degree from the Scuola Interpoltecnica di Dottorato (SIPD, Milano, Italy) in biomedical engineering, with a specialization in complex networks, intelligent systems and machine learning in biomedicine (2007-2009).
Sara Ciucci is currently undertaking her PhD in the group of Dr. Carlo Cannistraci. She is studying machine learning and network biology techniques for exploration and analysis of omics data. She obtained her Master’s degree from the University of Trento in Mathematics (Italy, 2014).
Publication
“Enlightening discriminative network functional modules behind Principal Component Analysis separation in differential-omic science studies”
Sara Ciucci, Yan Ge, Claudio Durán, Alessandra Palladini, Víctor Jiménez-Jiménez, Luisa María Martínez-Sánchez, Yuting Wang, Susanne Sales, Andrej Shevchenko, Steven W. Poser, Maik Herbig, Oliver Otto, Andreas Androutsellis-Theotokis, Jochen Guck, Mathias J. Gerl & Carlo Vittorio Cannistraci
Scientific Reports 7, Article number: 43946 (2017)
DOI: 10.1038/srep43946
http://www.nature.com/articles/srep43946
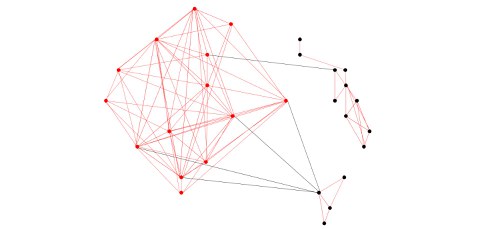
Exemplary illustration of combinatorial and multiscale
Media inquiries:
Franziska Clauß, M.A.
Press Officer
Tel.: +49 (0) 351 458 82065
The Biotechnology Center was founded in 2000 as a central scientific unit of the TU Dresden with the goal of combining modern approaches in molecular and cell biology with the traditionally strong engineering in Dresden. The BIOTEC plays a central role in the “Molecular Bioengineering and Regenerative Medicine” profile of the TU Dresden, fostering developments in the new field of Biotechnology/Biomedicine. The center focuses on cell biology, nanobiotechnology, and bioinformatics. https://www.biotec.tu-dresden.de