Description
Objective
The new RIAN methodology aims to employ artificial neural networks for the reliable identification of soil-hydraulic parameters from the outcome of dynamic laboratory outflow experiments. RIAN can be an alternative to the currently used inverse method (e.g. Levenberg-Marquardt) and features a number of advantages.
Procedure
RIAN comprises two independent moduls (Figure 1).
Module 1 In this module, a neural network is trained involving the following steps:
|
Modul 2 This module controls the routine applications of the trained network. The flow experiments must be carried out for the same experimental setup as used for training the network in Module 1. The measured time series of water contents, pressure heads and/or cumulated outflow are then presented to the trained network, which in turn identifies the corresponding soil-hydraulic parameters. |
The routines incorporated in Module 1 require considerable computing resources and can only be carried out on high performance machines. However, the tasks in Module 1 only have to be solved once for ensuring a continuous, straight-forward and easily repeatable application of Module 2, which can be performed on a normal PC.
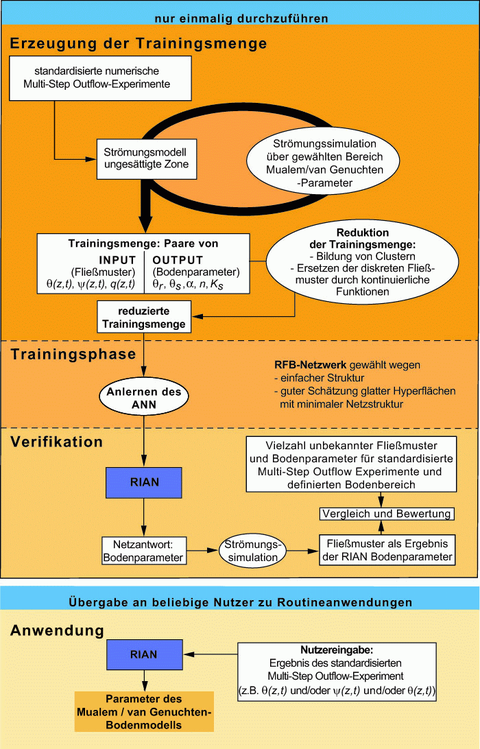
General structure of RIAN