Estimation of soil hydraulic parameters with hydrodynamic numerical methods and artifical neural networks
Despite considerable efforts, simulations of water and solute transport often fail to yield satisfactory results when applied to field scale processes. In this respect, the major difficulty is in the estimation of the soil-hydraulic parameters for describing the retention and conductivity characteristics of the soil. This research project aims to introduce Artificial Neural Networks (ANN) for deriving the soil-hydraulic characteristics from dynamic flow experiments together with a simplification and standardization of the laboratory experiments. The easy and straightforward application of the trained ANN can be seen as an effective alternative to the commonly used inverse method, which is not only a highly complex numerical procedure, but may also pose problems such as numerical errors and instabilities.
Teaching an ANN requires a database which includes all the information needed to describe the relationship between flow patterns and soil-hydraulic parameters. This database is generated numerically using a physically based model for the water transport and simulating a large number of flow scenarios for different soils and boundary conditions. In addition to these numerical experiments, dynamic laboratory experiments are carried out which cover typical flow scenarios such as infiltration, evaporation, and percolation. The outcome of these experiments is then presented to both the inverse method and the trained ANN. This comparison finally allows the assessment of the overall reliability of the new methodology.
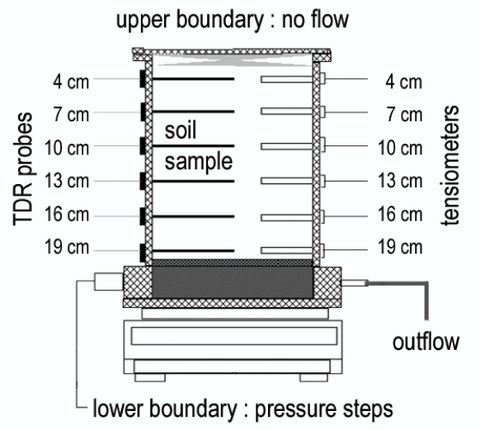
experiment set-up of RIAN
A comparison with the inverse method (Annealing-Simplex) reveals a similarly high predictive accuracy of both approaches for synthetic and real world data. Nonetheless, the inverse method showed a dependency on initial parameter estimates which is not the case with the RBF network. With regard to computational or manpower requirements, the network training is moderately more demanding than the inverse technique, whereas the absolutely stable and simple network application requires negligible resources. Thus, for individual applications, the Annealing-Simplex method is slightly superior to the network approach. However, the RBF network only has to be trained once and, subsequently, can be applied easily and effortlessly upon any number of laboratory experiments when employing standardised experimental setups.
Duration: März 2001 - Dez. 2005
sponsored by: DFG