Description
The complex demands placed on hydrologic / hydraulic models and their mathematical sophistication contrast strongly with the operational necessity for the forecast systems to be robust, fast and straightforward. A way out of this dilemma is the combining of process models with specific task adapted artificial neural networks. The PAI-OFF methodology (Process Modelling and Artificial Intelligence for Online Flood forecasting) – developed at the Institute for Hydrology and Meteorology at the TU Dresden – can exploit the benefits of the latest hydro-dynamic numerical models for a real time robust and straightforward operation within the framework of an online operation system for flood forecasting.
With the help of observation data and run-off relevant catchment characteristics, we establish a process oriented, coupled hydrologic / hydraulic model of the catchment area. On the basis of region specific meteorological characteristics, the model calculates all the feasible constellations and scenarios which would be physically possible in terms of flood formation.
A task specific learning strategy transfers the hydrologic/hydraulic characteristics of the catchment area to the artificial neural network, incorporating the flood relevant initial state of the catchment. In this way, PAI –OFF overcomes the major restriction of scarce and limited observation (learning) data, which - in the past - was the main reason for failure when attempting to use ANN for flood forecasting.
The aforementioned steps are carried out once only for each specific catchment area. Subsequently, PAI-OFF can be employed routinely and straightforwardly – without hassle – as a valuable tool for flood forecasting.
The general advantages of artificial neural networks – such as simplicity, robustness, speed and efficiency, the ability to adapt quickly to the catchment area’s behaviour and / or to consider the effects of changes e.g. in land use(learning by doing) – can all be fully exploited and use all the benefits of comprehensive, state of the art hydrologic / hydraulic modelling.
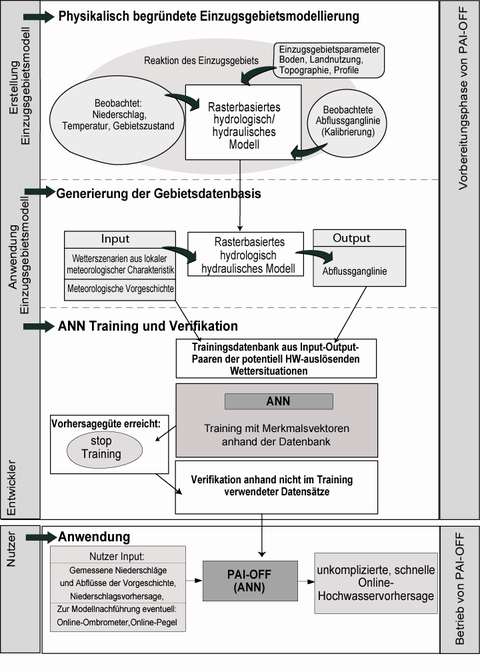
Arbeitsschritte
With respect to PAI-OFF, any type of model can be employed for the hydrologic modelling of the catchment area in question. As a result of a comprehensive analysis, we decided upon a WaSIM-ETH model, which efficiently reflects soil moisture distribution with the help of the Richard’s equation (GURTZ et al. 2000). The gauged rainfall data is projected onto the investigated area by using external drift kriging (temperature, precipitation) and ordinary kriging (air humidity, wind speed, global radiation).
The coupling of the rainfall-runoff model with flood routing models recommends a flexible use of 2 different approaches for the portrayal of flood wave propagation. Along these lines, in steep and narrow river reaches a translation diffusion approach is used (WaSIM-ETH) whilst the areas featuring back water effects are modelled by the one-dimensional hydrodynamic numerical model HEC-RAS.
Because the pre-flood hydrologic phenomena strongly influence flood formation processes, the proposed PAI-OFF approach incorporates information about the foregoing course of events. It does this by using a phenomenon specific feature vector.
Using the meteorological input parameters provided by the regional model of the German Weather Service subsequently allows the problem-specific artificial neural network PAI-OFF to straightforwardly and reliably predict the discharge at the selected river-gauge.