Efficient Numerical Treatment of Polymorphic Uncertainty by Hierarchical Tensors
A continuation of the topic is currently taking place in the second project phase.
-
Goal 1: Combined Model Reduction and Tensor Compression
-
Goal 2: Extraction of Quantities of Interest from Low Rank Tensors
-
Goal 3: A posteriori Reliability Check
The PolyH surrogate model was developed based on Chebyshef polynomials and the Hierarchical Tree Tensor formats. Three options to construct this surrogate model were investigated. First, construction as a solution to a large linear system which originates from a PDE. Second, by the use of generalized cross approximation for black box models. Third, using general non-linear optimization if only data is given.
To make polymorphic uncertainty quantification feasible, methods based on PolyH to avoid sampling for the stochastic part and thus reduces the computational costs by several magnitudes were developed. Two options for the combination of PolyH and POD were explored for neo-hookean elastic models. First we compared a PolyH model which was build upon the full model to the POD model and found, that the accuracy reached by POD was preserved in the PolyH model. Second, we used the PolyH model to find snapshots for POD, which increased the performance of POD.
Essential project findings
- Reduction of computational cost by several magnitudes for polymorphic uncertainty quantification
- PolyH based on POD was constructed
- In the reverse direction PolyH was used to choose snapshots for POD
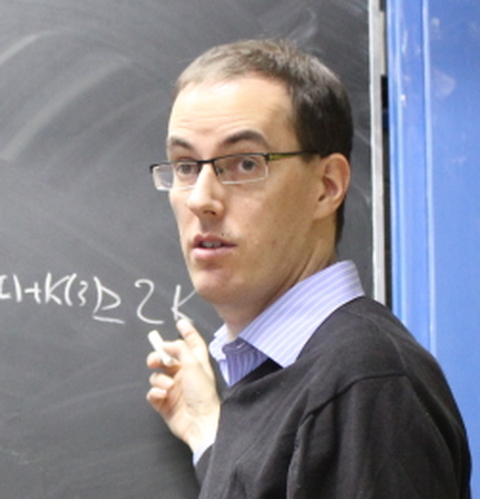
Prof. Grasedyck
Prof. Dr. Lars Grasedyck
RWTH Aachen
Institut für Geometrie und praktische Mathematik (IGPM)
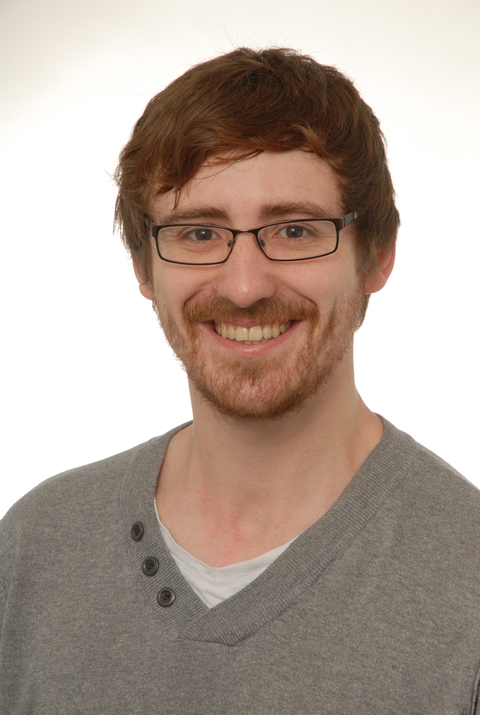
Dieter Moser
Dieter Moser
RWTH Aachen
Institut für Geometrie und praktische Mathematik (IGPM)
Kastian, Steffen, Moser, Dieter et al. "A two-stage surrogate model for Neo-Hookean problems based on adaptive proper orthogonal decomposition and hierarchical tensor approximation." Computer Methods in Applied Mechanics and Engineering 372 (2020): 113368.