Structural design of reinforced concrete based on multiscale modelling and polymorphic uncertainty
A continuation of the topic is currently taking place in the second project phase.
The realistic modelling of reinforced concrete structures is essential for their numerical design and is mainly characterized by the mechanical model and the consideration of the available data at hand by an adequate uncertainty model. The project captures the two main aspects of numerical simulation6 by linking and extending the description of uncertainty and the mechanical modelling in a profound manner. Hence, the application of the methods and approaches, which are developed within this project, provides the means to investigate and to evaluate the influence of the uncertainty, bridging the scales from mesoscopic uncertainty to macroscopic uncertainty, onto the overall structural behavior of reinforced concrete structures.
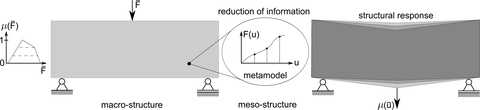
Schematic sketch of the computation of the macroscopic structural response, considering uncertain data on the macro-struture (exemplary modelled as fuzzy quantities) and the reduced uncertain response at the meso-structure
Following objectives are focussed:
-
developing multi-scale methods and numerical approaches for the design of RC structures under consideration of their polymorphic uncertainty of data and material heterogeneity
-
evaluation of the influence and the consequence of polymorphic uncertainty at the mesoscale of RC structures on the overall macroscopic structural behaviour
-
developing a partial spatial homogenization approach and investigating the admissibility of a local computational homogenization
-
developing admissible methods for generating RVEs within partially heterogeneous solids
Summary
For the numerical design of structures, realistic modeling is essential. This is basically characterized by the mechanical description of the available data by means of an adequate uncertainty model. The application of the developed methods in the numerical design considering the scale transition of uncertainties investigates and evaluates the influence of mesoscale uncertain quantities to macroscopic quantities of concrete structures.
An automated generation algorithm of a representative volume element (RVE) includes the orientation and size of aggregates and air voids. Significant uncertain geometry parameters are the underlying grading curve, the volume ratio of aggregate to matrix, and the spatial distribution and orientation of the aggregates and air voids, respectively. The damage to the matrix material is numerically modeled by an eigen-erosion approach, and the parameters of the damage formulation are assumed to be fuzzy or interval quantities. The uncertain material parameters are quantified by a developed inverse uncertainty quantification based on real-world concrete test data. Assuming that the choice of uncertainty models depends on the data size and quality, the UQ methods are developed for interval quantities. Both fuzzy and fuzzy-probability based random variables can be composed out of probabilistically or possibilistically assessed interval variables.
Numerical consideration of the mesoscopic mechanical behavior of the RVE is approximated using numerical material testing (NMT) and recurrent neural networks (RNN). A set of displacement boundary conditions and the resulting stress responses serve as the data basis of the RNN. The RNN is constructed by long-short term memory (LSTM) cells, enabling time dependent input (strains) and predicted output (stresses) variables. Analogous to the uncertainty quantification scheme, a separate RNN is applied for interval-valued stress responses for each interval boundary. At each macroscopic integration point, the material tangent is equal to the strain-dependent gradient of the RNN output quantities, making the RNN applicable as constitutive description in conventional FE solvers. At the structural level, the considering of uncertain mesoscopic quantities, uncertain structural responses are determined for deterministic macroscopic boundary conditions, which result from the prescribed uncertain on the mesoscale.
Essential project findings
- automated structure generator of an RVE for concrete, with uncertain geometrical and mechanical parameters
- framework for spatial homogenization based on numerical material tests of the RVE and recurrent neural networks
- approximation of stress-strain relations with long-short term memory cells
- gradient of LSTM output as macroscopic material tangent
-
Leichsenring, F.; Graf. W.; Kaliske, M.:
Surrogate Model based Structural Analysis of reinforced concrete structures with Polymorphic Uncertainties. 3rd International Conference on Uncertainty Quantification in Computational Sciences and Engineering (UNCECOMP),
Crete, 2019 -
Leichsenring, F.; Graf. W.; Kaliske, M.:
Modelling of polymorphic uncertainty in the mesoscopic scale of reinforced concrete structures. 13th International Conference on Applications of Statistics and Probability in Civil Engineering (ICASP13), Seoul National University, 2019 -
Leichsenring, F; Fuchs, A.; Graf. W.; Kaliske, M.:
Application of Recurrent Neural Networks in Structural Analysis of reinforced concrete structures considering polymorphic uncertainty. 90th Annual Meeting of the International Association of Applied Mathematics and Mechanics (GAMM),
TU Wien, 2019 -
Kremer, K.; Edler, P.; Miska, N.; Leichsenring, F.; Balzani, D.; Freitag, S.; Graf, W.; Kaliske, M.; Meschke, G.:
Modeling of structures with polymorphic uncertainties at different length scales.
Surveys for Applied Mathematics and Mechanics (GAMM-Mitteilungen), 2019, Link - Graf, W.; Richter, J.; Götz, M.; Kaliske, M.:
Numerical analysis of reinforced concrete based on multiscalemodelling and polymorphic uncertain data. In: Beck, A.T. (ed.),3thInternational Conference on Vulnerability andRisk Analysis and Management (ICVRAM) & 7thInternational Symposium on Uncertainty Modelling and Analysis (ISUMA), Florianopolis & University of São Paulo, 2018 - Leichsenring, F.; Jenkel, C.; Graf, W.; Kaliske, M.:
Numerical simulation of wooden structures with polymorphicuncertainty in material parameters, special issue onComputing with polymorphic uncertain data (REC 2016),International Journal of Reliability and Safety,Vol. 12 (2018) No. 1/2, pp. 24-45DOI: 10.1504/IJRS.2018.10013787 - Graf, W.; Götz, M.; Kaliske, M.:
Computational framework for design of structures with polymorphic uncertaindata. In: Bucher, C. et al. (eds.), Proceedings 12th ICOSSAR, pp. 1163-1173, TU Vienna, 2017 - Götz, M.; Serafinska, A.; Leichsenring, F.; Graf, W.; Kaliske, M.:
Multi objective optimization considering poly-morphic uncertainty. In:2ndInternational Conference Uncertainty Quantification in Computational Sciences andEngineering (UNCECOMP), abstract (online available), Rhodos, 2017